Looking ahead to ML-powered journalism
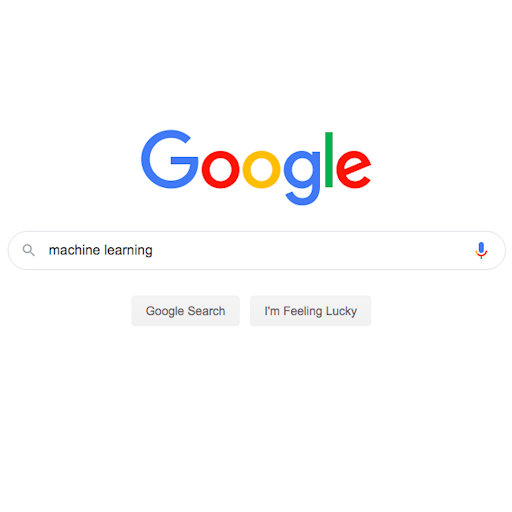
Key learnings and recommended resources to deepen your ML knowledge.
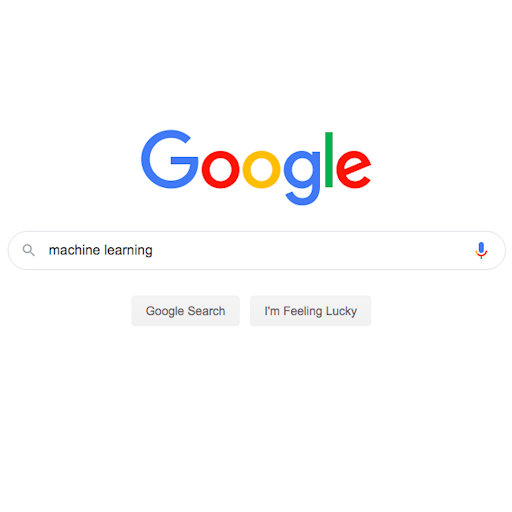
Moving the next steps
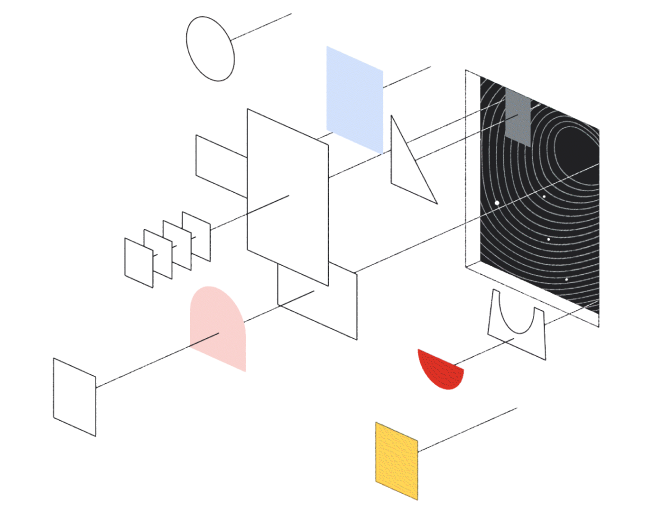
You have reached the end of this crash course on classifying images with machine learning. You should now have a better understanding of how machine learning works in practice, as well as what its power and limitations are.
If you have previously taken our Introduction to Machine Learning, you now have the perfect toolkit to understand how to enhance your reporting with machine learning and how you can make the most, in a responsible way, of the power offered by these technologies.
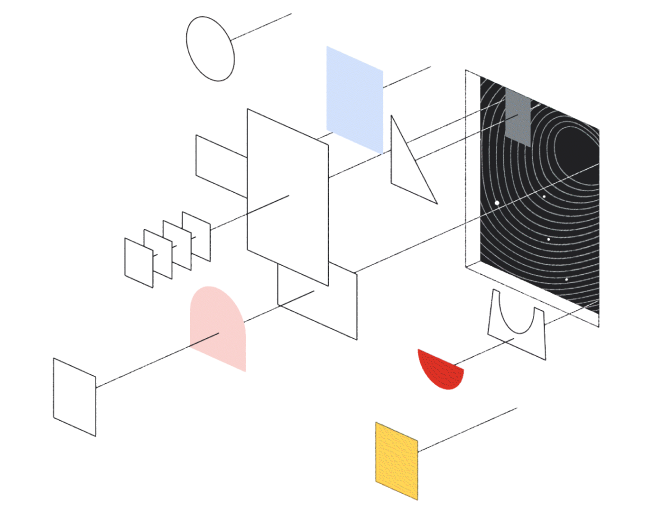
Summary of the training steps
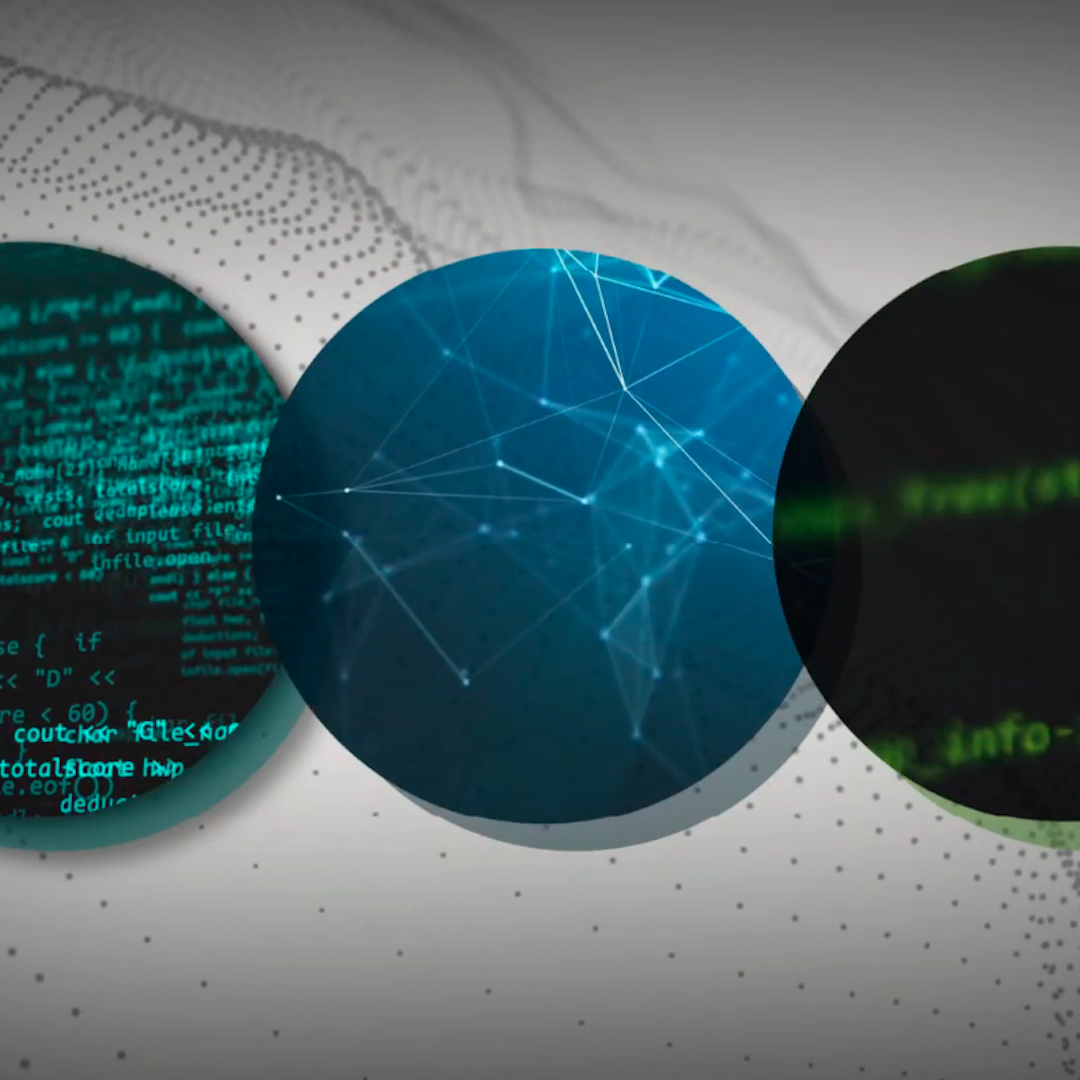
Let's recap all the steps involved in training a machine learning model:
- Reflect on your use case and consider whether ML can be (part of) the solution to the problem you are trying to solve.
- Source the data you need and take every possible step to minimise the potential impact of biases in the data.
- Clean and prepare the data so that it contains the right balance of information the model needs to learn from.
- Choose the algorithm that is best suited to meet the goals of your use case and the features of your training data.
- Upload the dataset you prepared to the algorithm of your choice and wait for it to learn.
- Evaluate the results and decide if they are good enough to use the model for your journalistic purpose. If not, repeat.
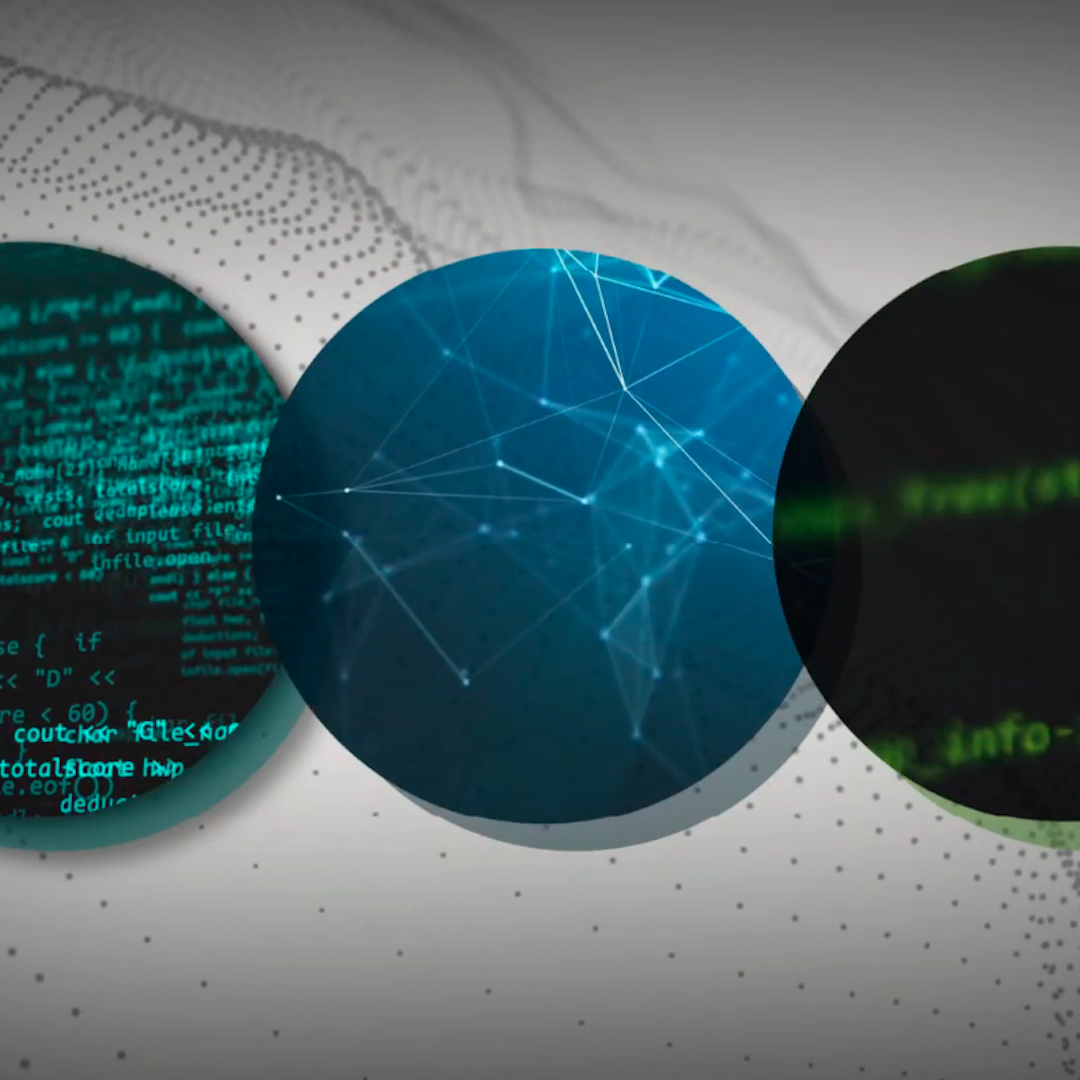
Want to learn more?
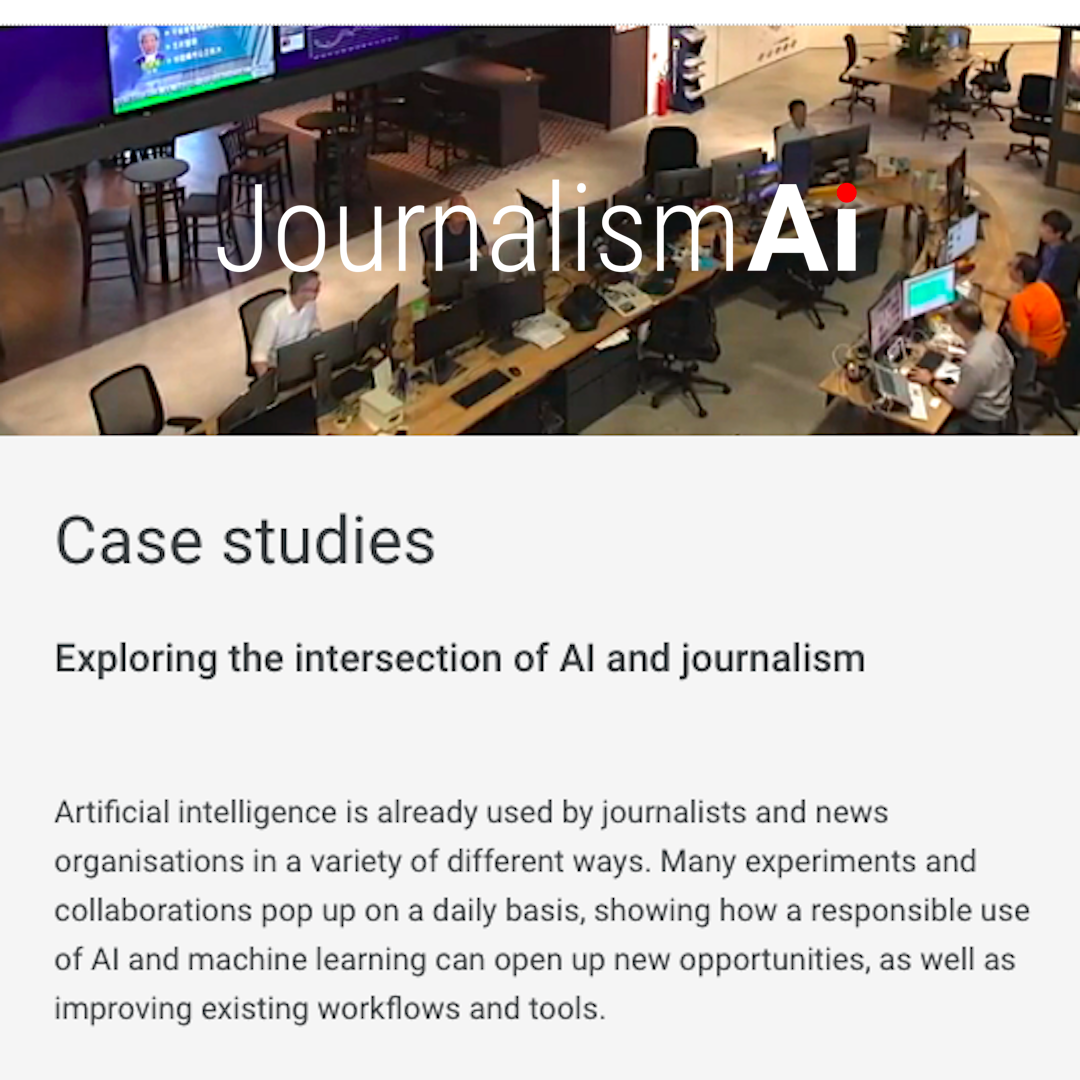
In this crash course, we learned how machine learning can be used to classify images in the context of an investigation, but there are many other journalistic use cases. Find a few examples in this handy collection by the Quartz AI Studio that highlights instances of "how you might feel when machine learning can help".
If you want to know more about how news organisations use machine learning and other AI-powered technologies, browse the ever-growing library of case studies curated by the JournalismAI team.
And never forget to carefully reflect on how to identify potential biases in your data and make sure that they are not replicated and multiplied by your ML model. Check out this Google Cloud guide on Inclusive ML to learn more.
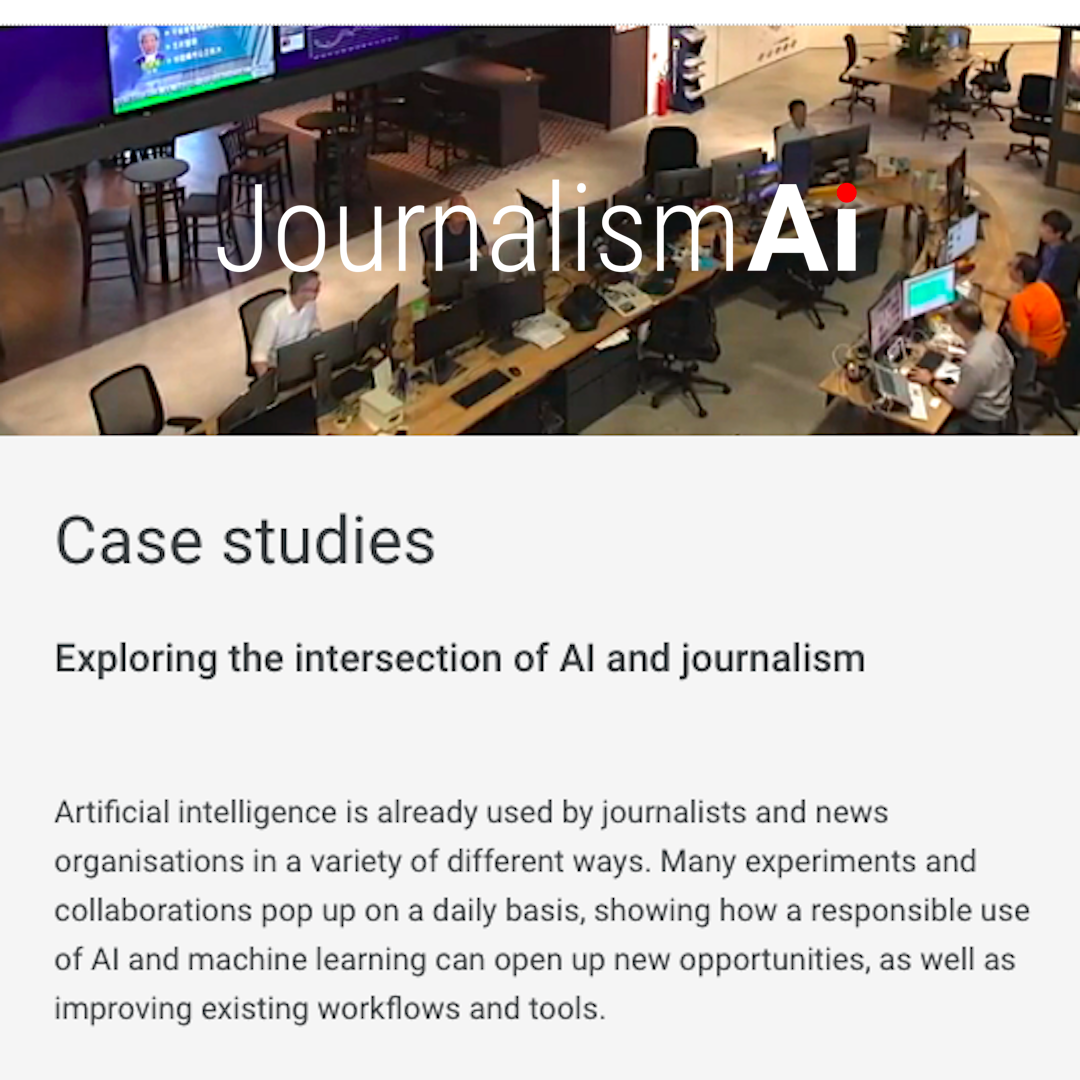
Credits
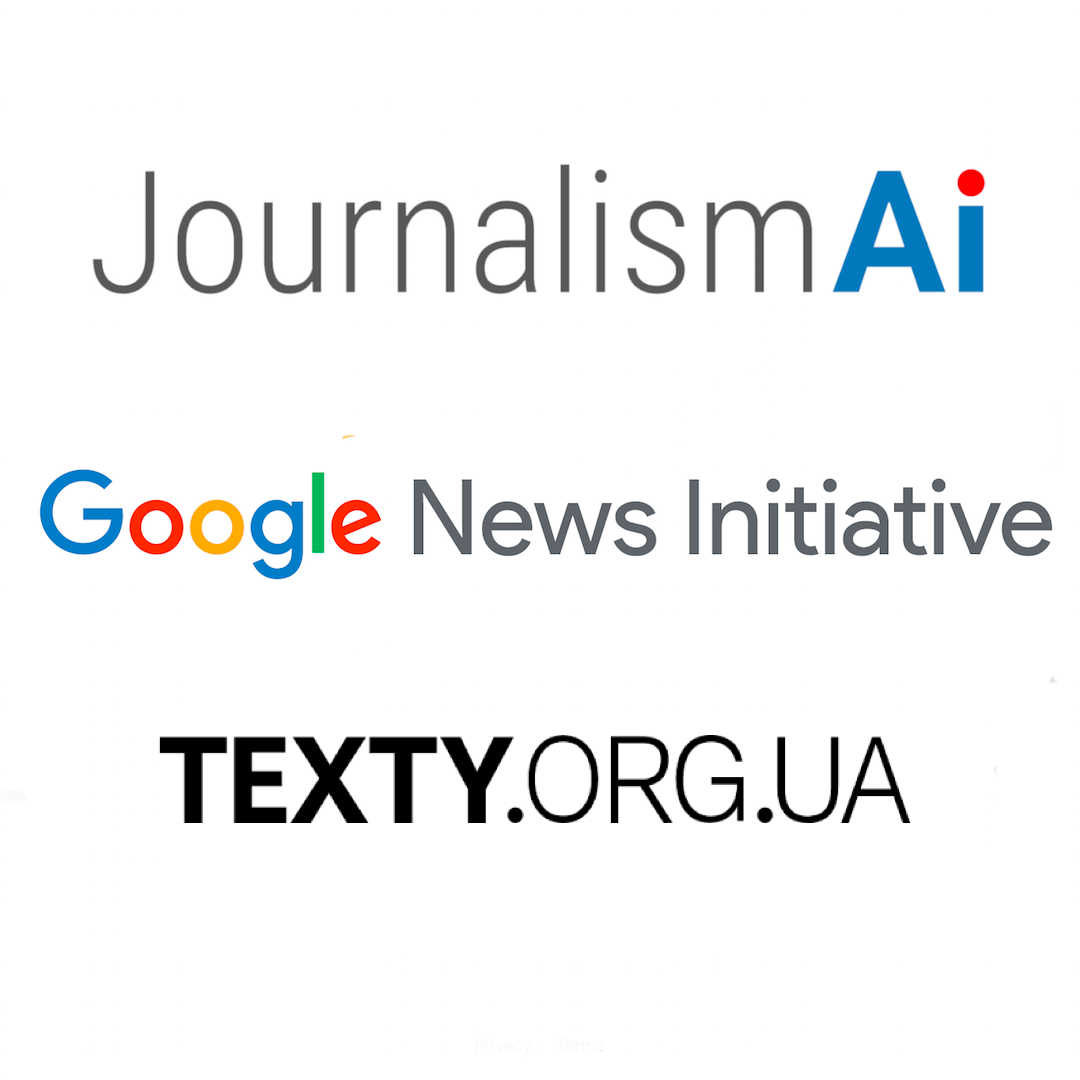
This course was developed by JournalismAI in collaboration with Anatoliy Bondarenko and his team at Texty
Texty is a Ukrainian data journalism agency that promotes transparency and accountability by developing high-quality journalism and data journalism, which includes analysis and presentation of big data in an interesting and comprehensive way.
JournalismAI is a project of POLIS – the journalism think-tank at the London School of Economics and Political Science – and it's funded by the Google News Initiative.
Special thanks to Agnes Stenbom, Fabienne Meijer, Florencia Coelho, and Jarno Koponen, for their precious feedback during the development of the course.
Sign up to the JournalismAI newsletter to stay informed about project activities.
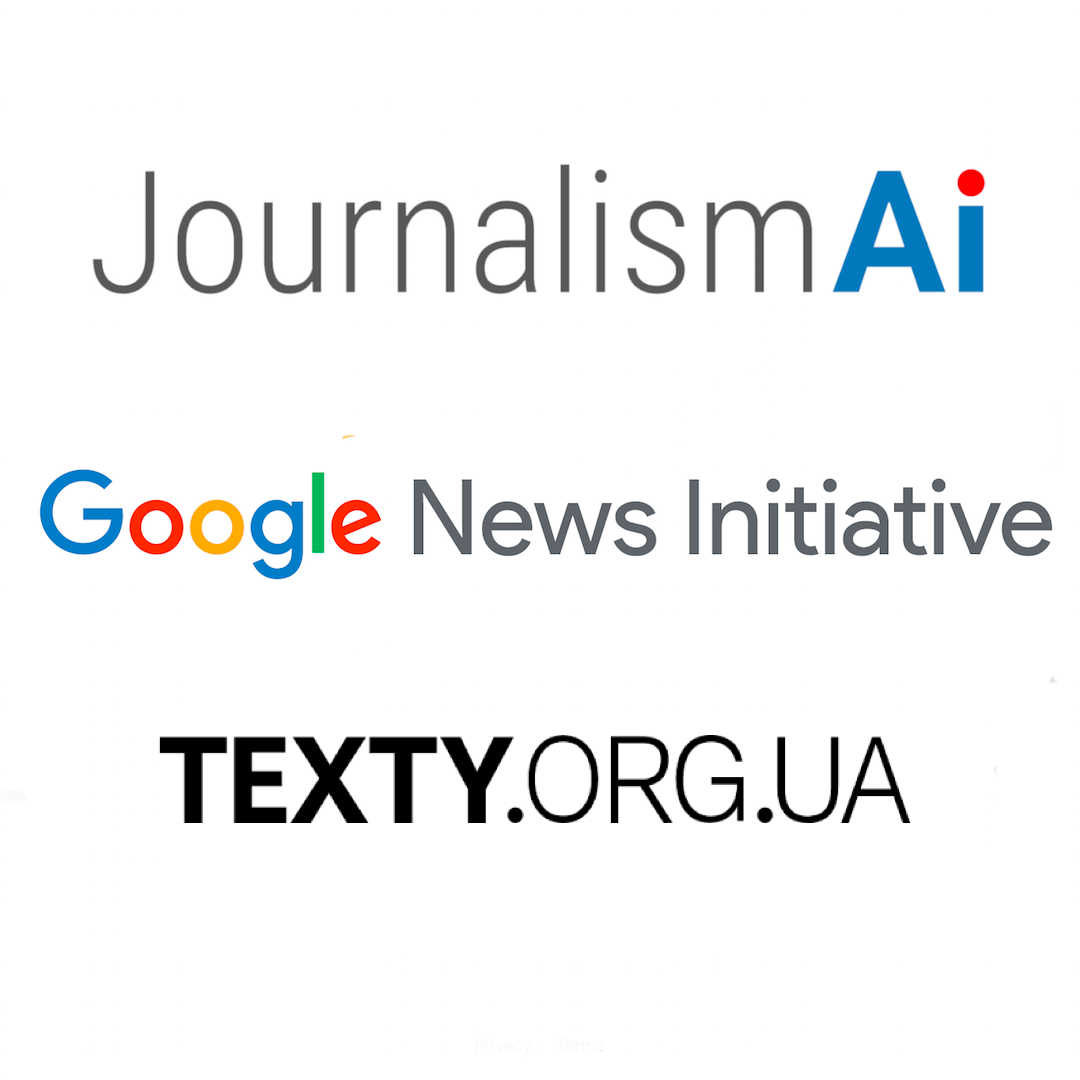
-
Guide de démarrage rapide pour la recherche d’ensembles de données.
LeçonEffectuez une recherche parmi des millions d’ensembles de données sur le web. -
Augmenter vos revenus issus des annonces numériques avec Google Ad Manager
LeçonLancez-vous sur Google Ad Manager pour augmenter vos revenus issus des annonces numériques. -
Apprenez-en plus sur vos audiences avec des enquêtes
LeçonComprenez vos audiences, leurs habitudes et ce qui compte pour elles avec des enquêtes.