Investigating stories with Machine Learning
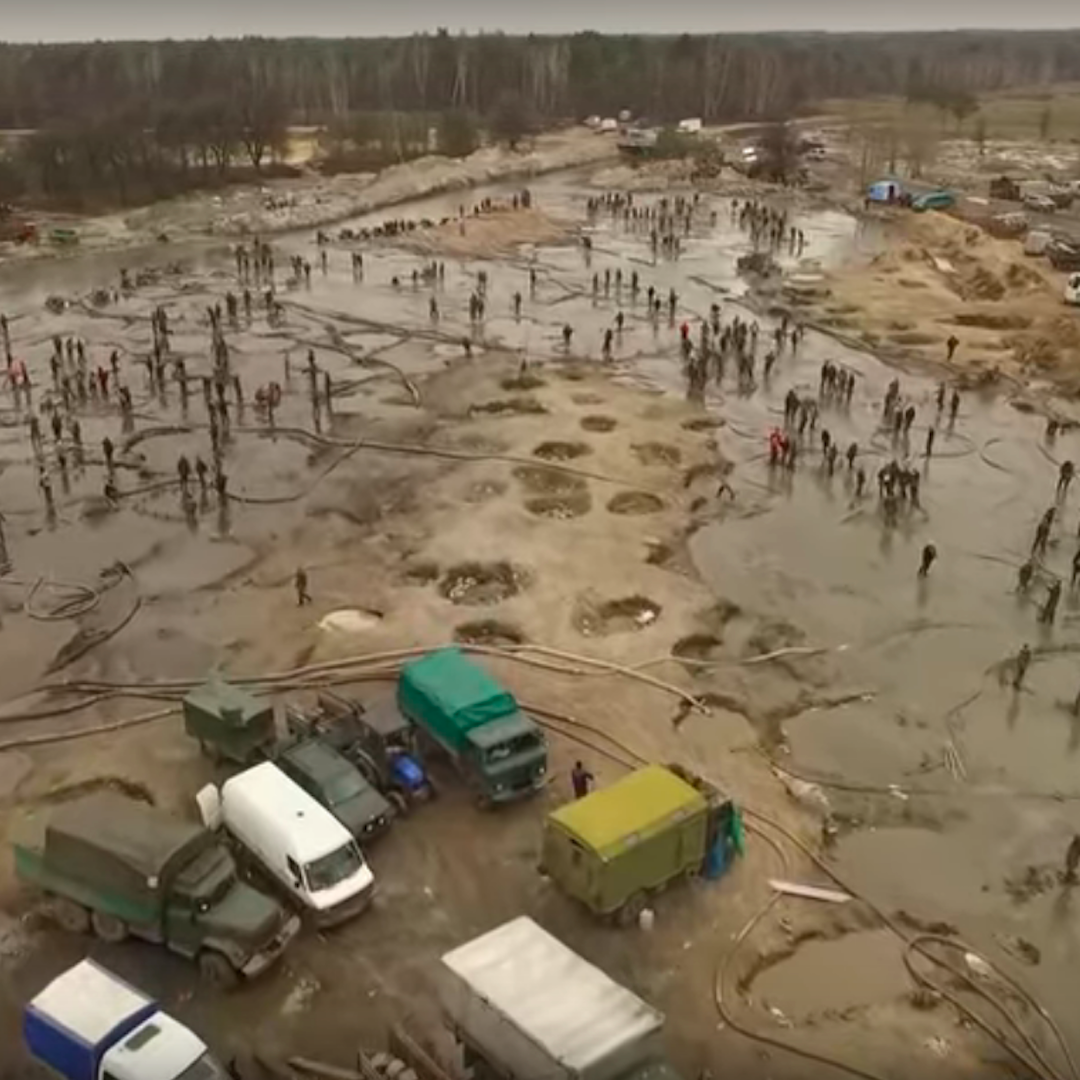
How you can use Machine Learning in your reporting
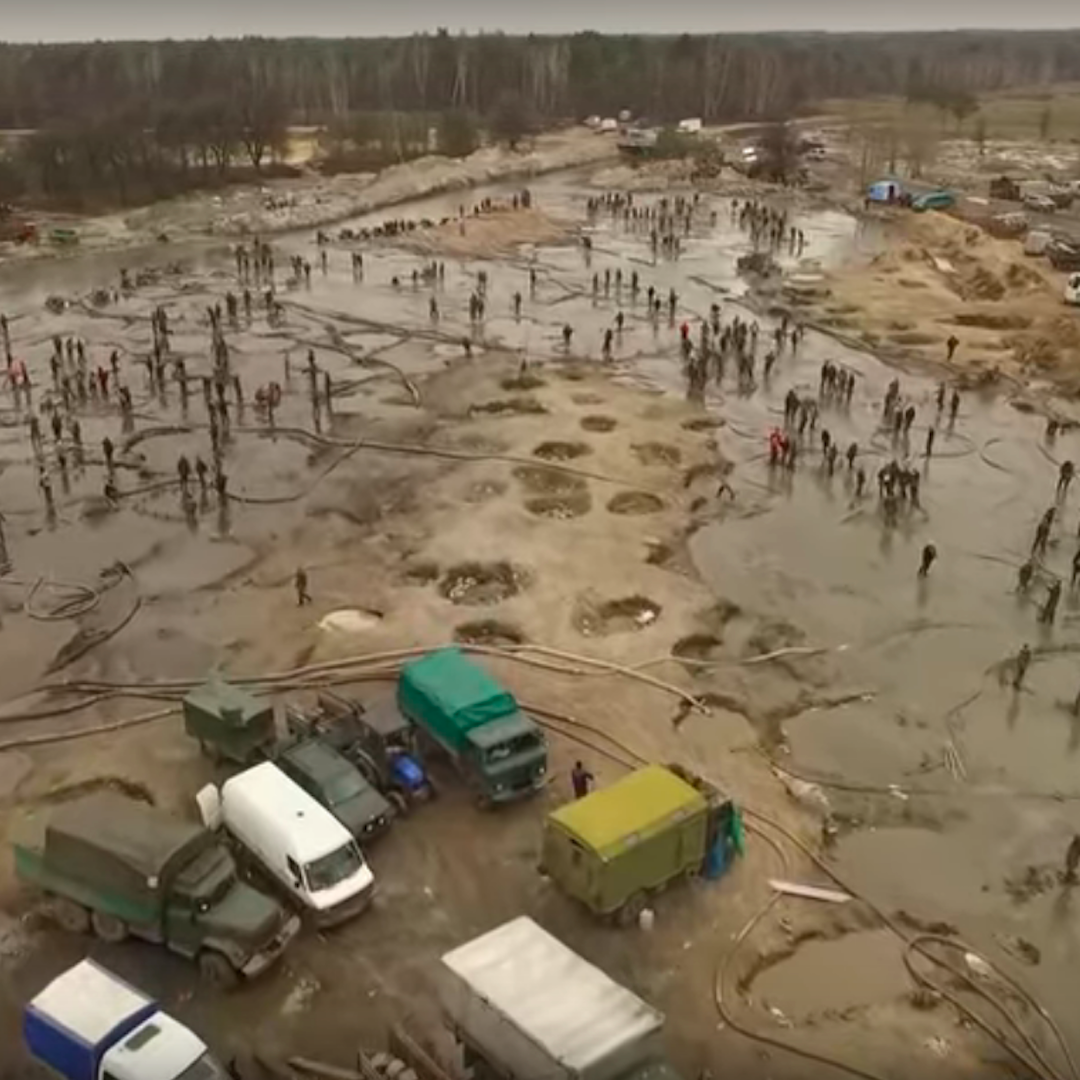
Machine Learning for investigations: a case study
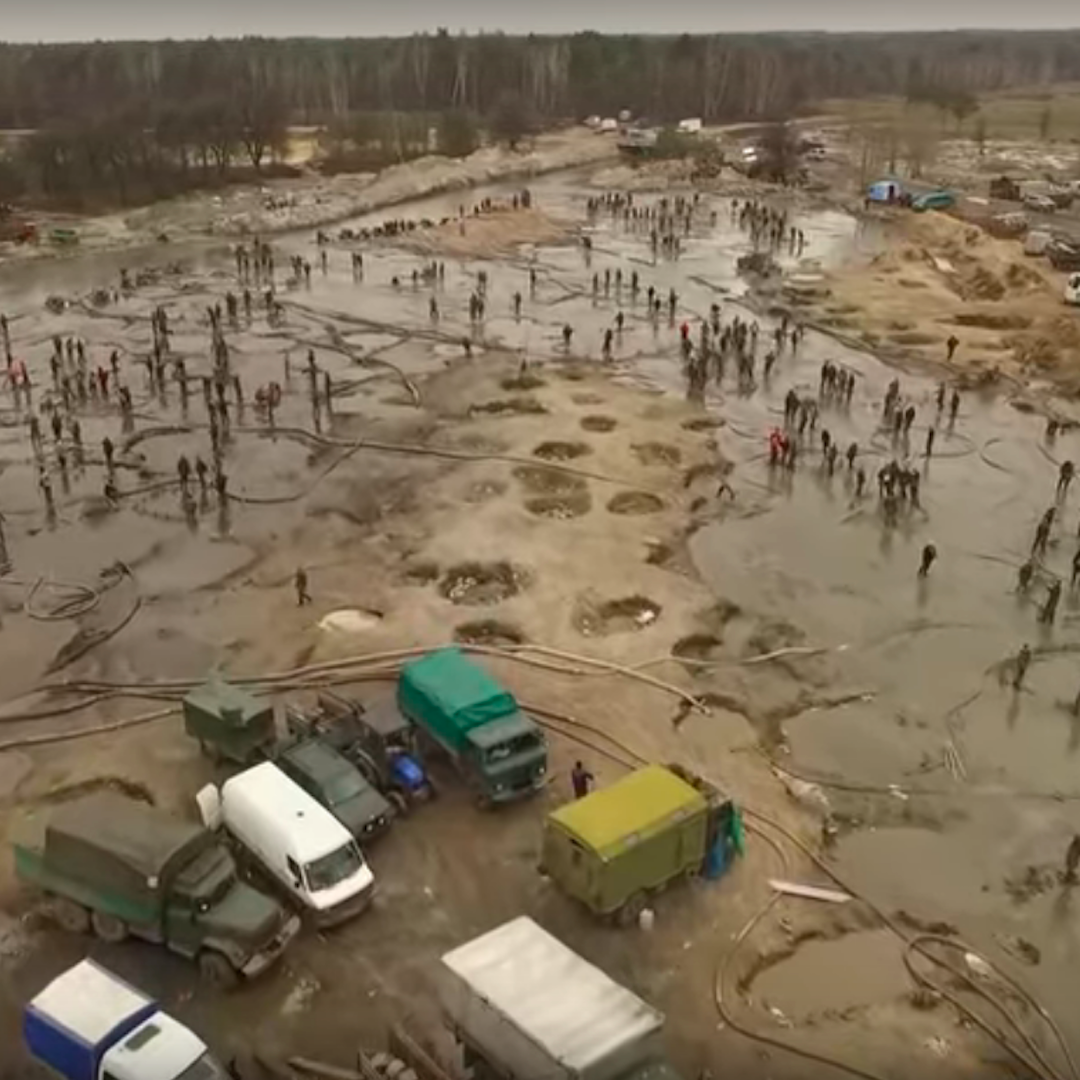
In 2010, the price of amber on the global market started to surge. Due to the high demand, in the following years parts of north-western Ukraine, rich in amber, attracted foreign and local interest and became the scene of an illegal "amber rush", a new "Wild West".
Hundreds of hectares of forests and agricultural land were turned into a lifeless moon landscape, with the most intense mining activity taking place between 2014 and 2016 but continuing over the following years.
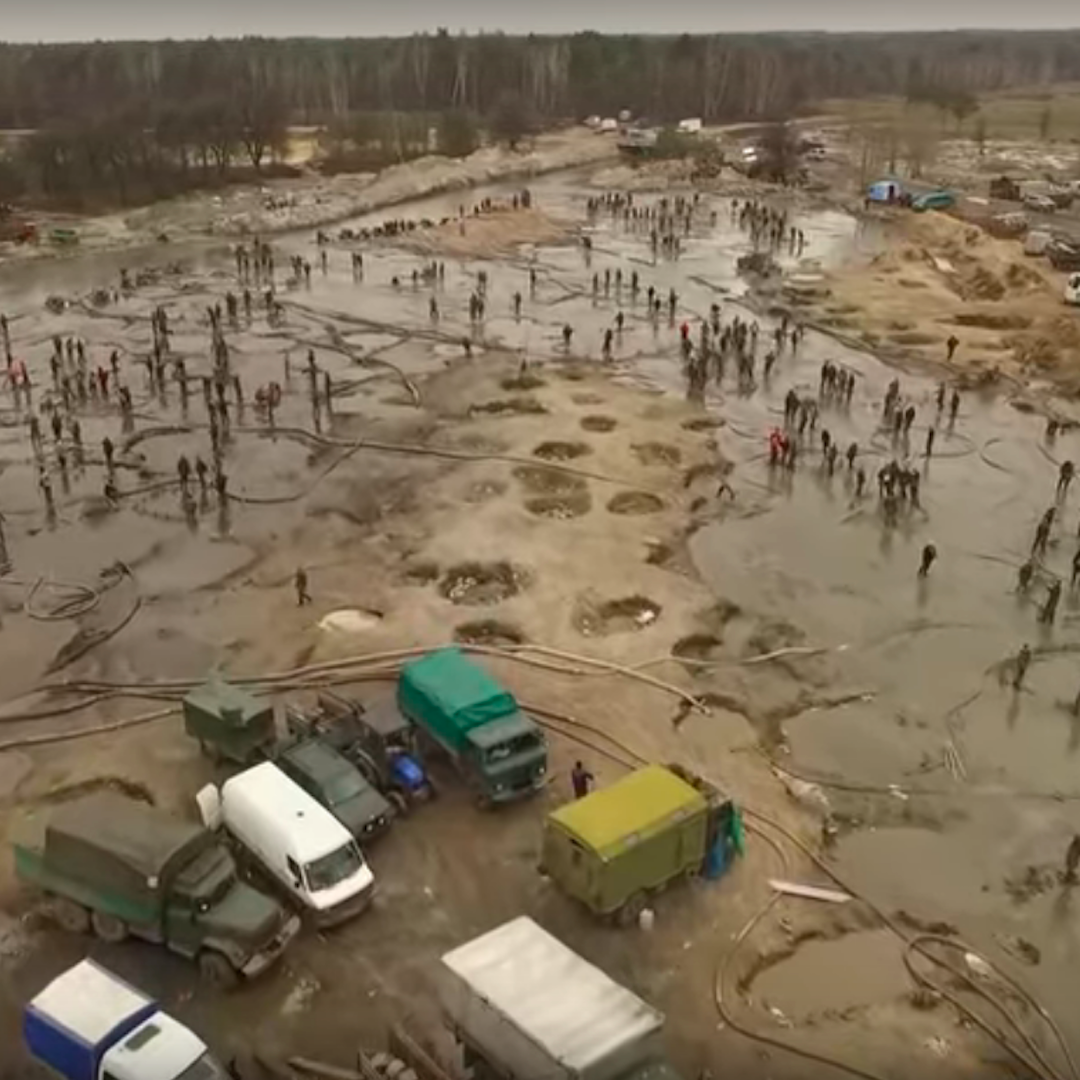
Leprosy of the Land, an investigation by Texty
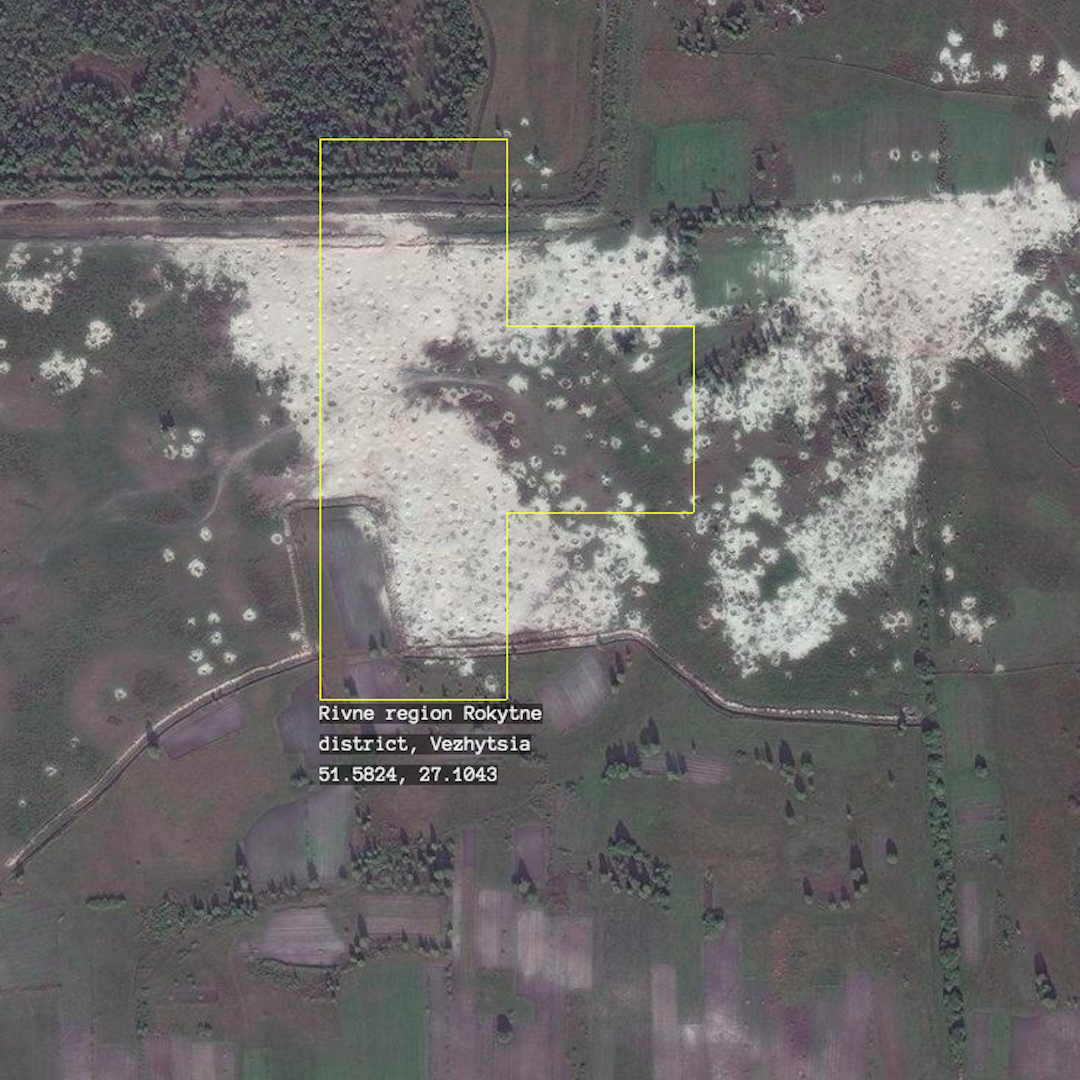
In 2018, Ukrainian data journalism agency Texty published Leprosy of the Land, an investigation in which they used machine learning techniques to detect cases of illegal amber mining across Ukraine.
First, an algorithm divided sections of satellite images into visually uniform subsections. So if an image was half green forest and half dirt field, it would split the image into those two subsections.
Another algorithm found which subsections most resembled the existing examples of amber mining, which have a distinctive pockmark-like pattern of holes in the ground.
Finally, the journalists examined the examples the algorithm found, to make sure that what it thought looked like amber mining wasn't actually something else, like deforestation.
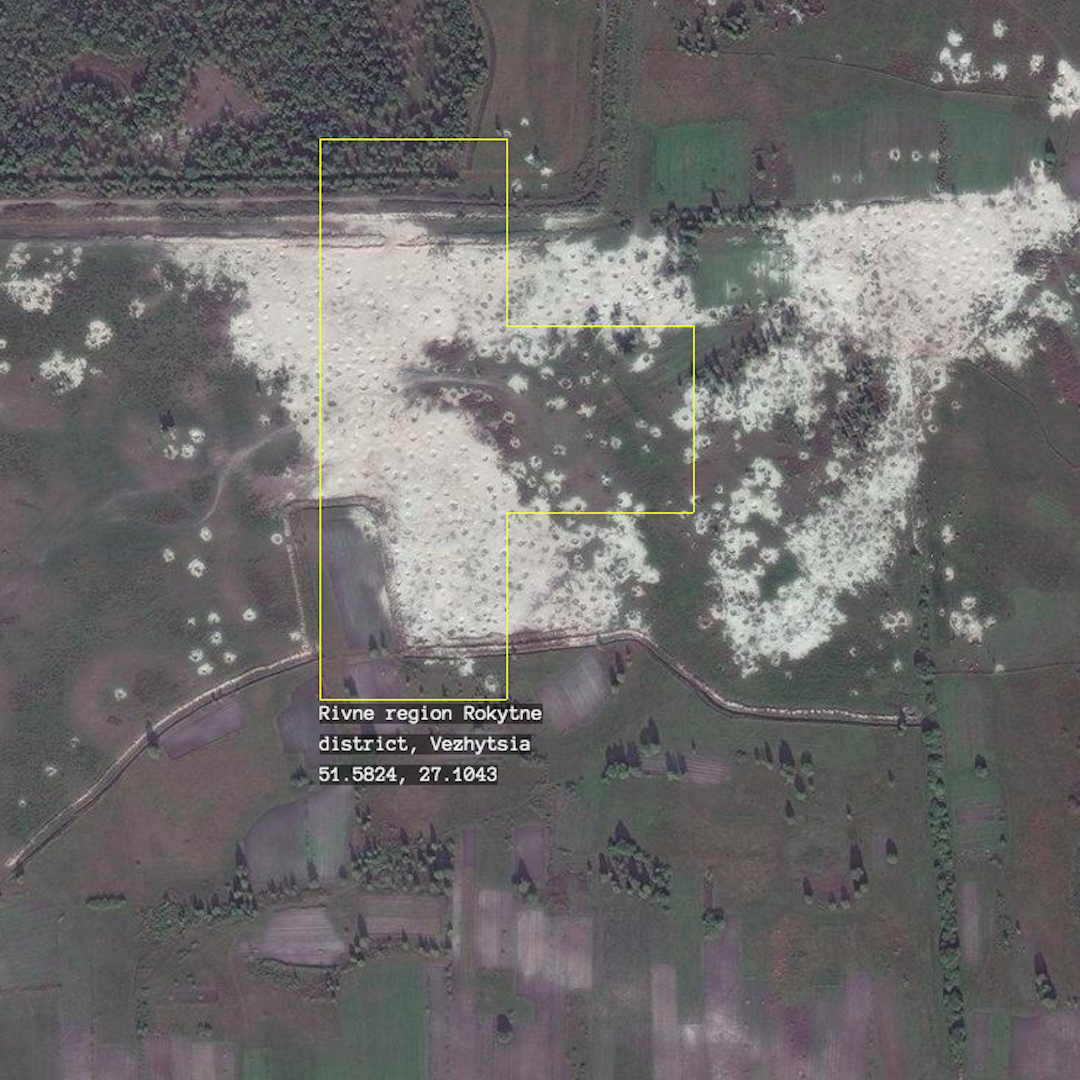
Finding examples of illegal amber mining
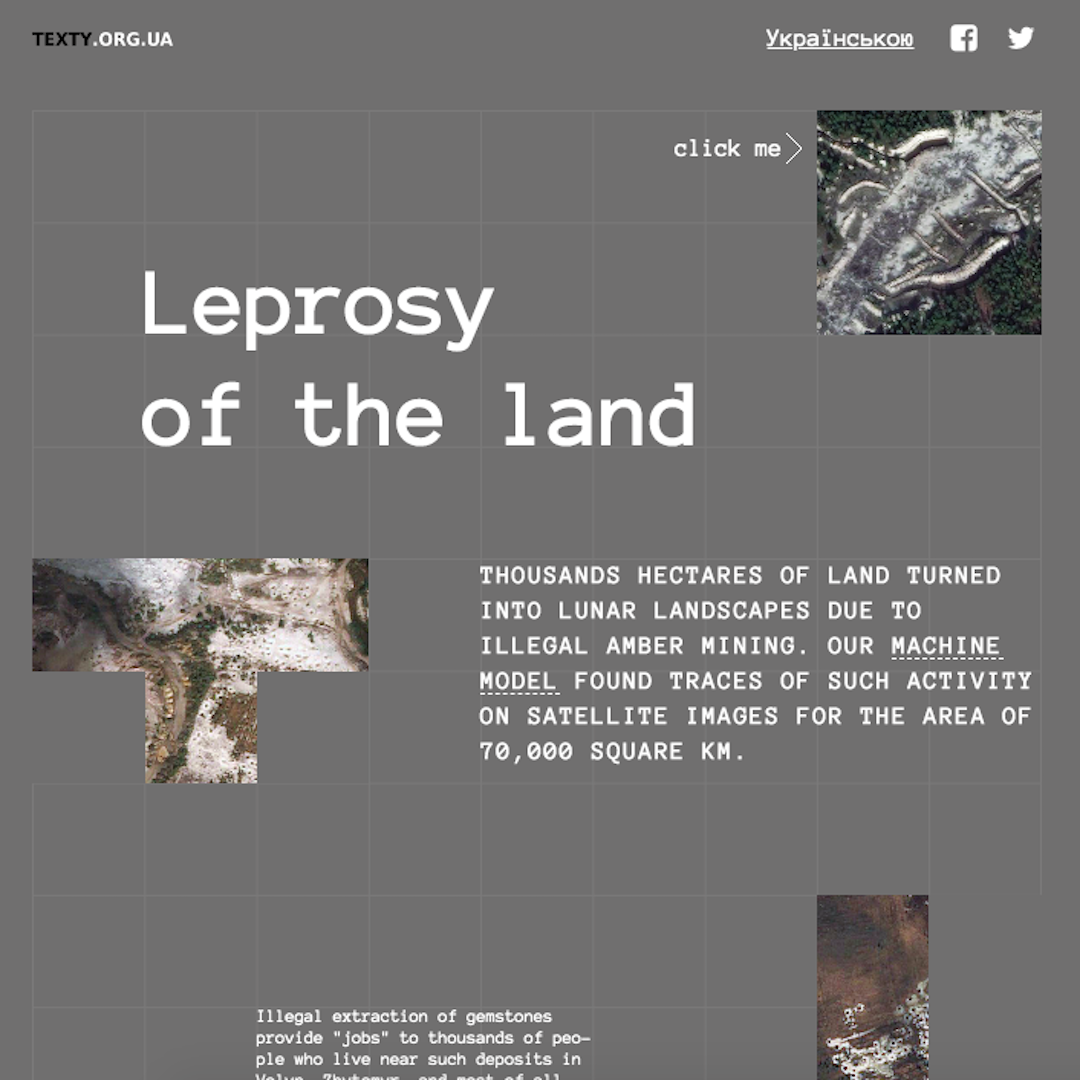
In this course, we will focus on the methods used by Texty to train an algorithm to recognise visual examples of illegal amber mining in a huge amount of satellite images, previously divided in subsections by another algorithm.
As mentioned in the first lesson, this means we will experiment with supervised learning. You will learn how the algorithm can learn from labelled examples to recognise the same pattern in images it has never seen before.
You will also learn how you can replicate the process for your own stories: from finding the examples you need, to training a machine learning model to recognise what you are looking for, and then to testing and evaluating the model to make sure it provides reliable results.
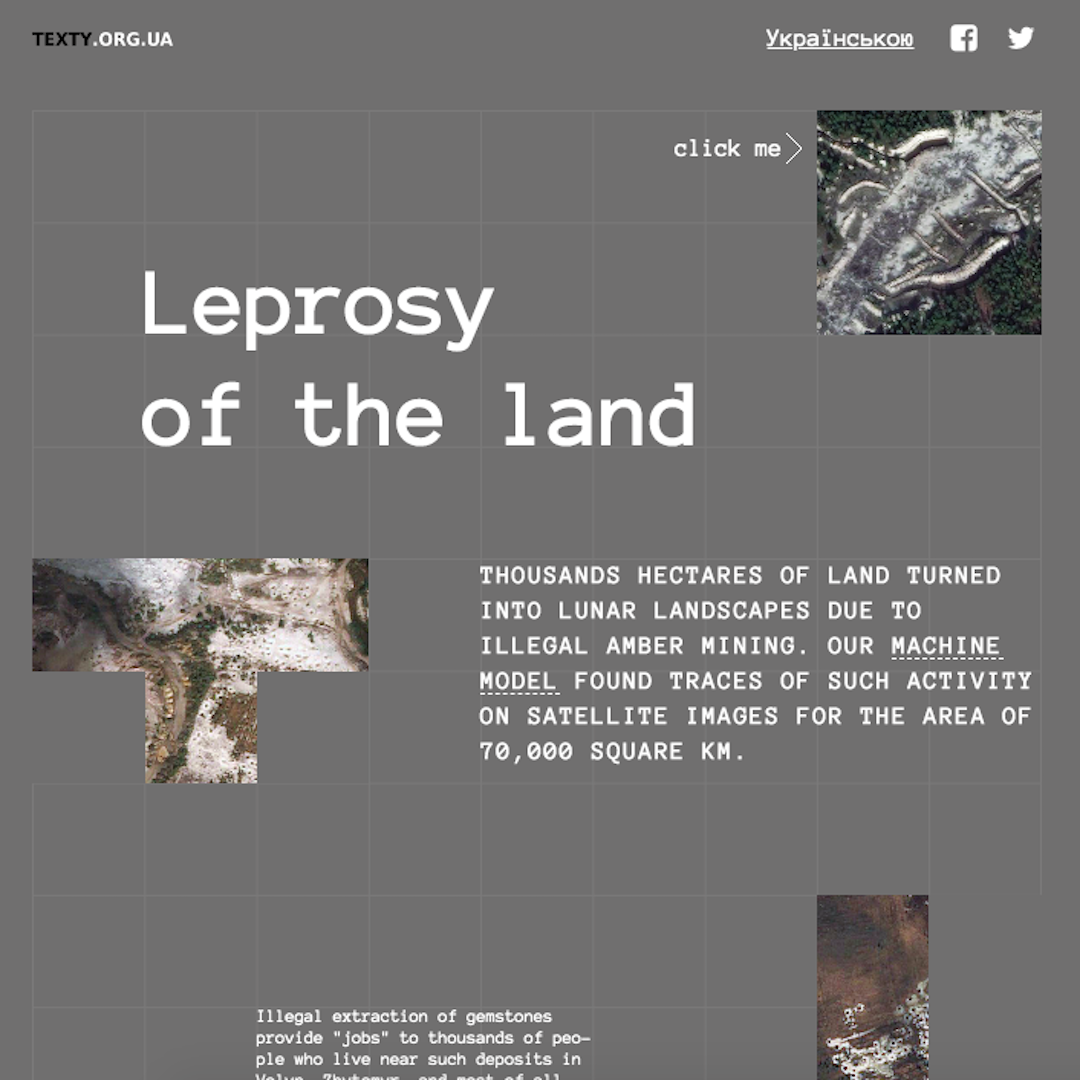
Is ML the right tool for this problem?
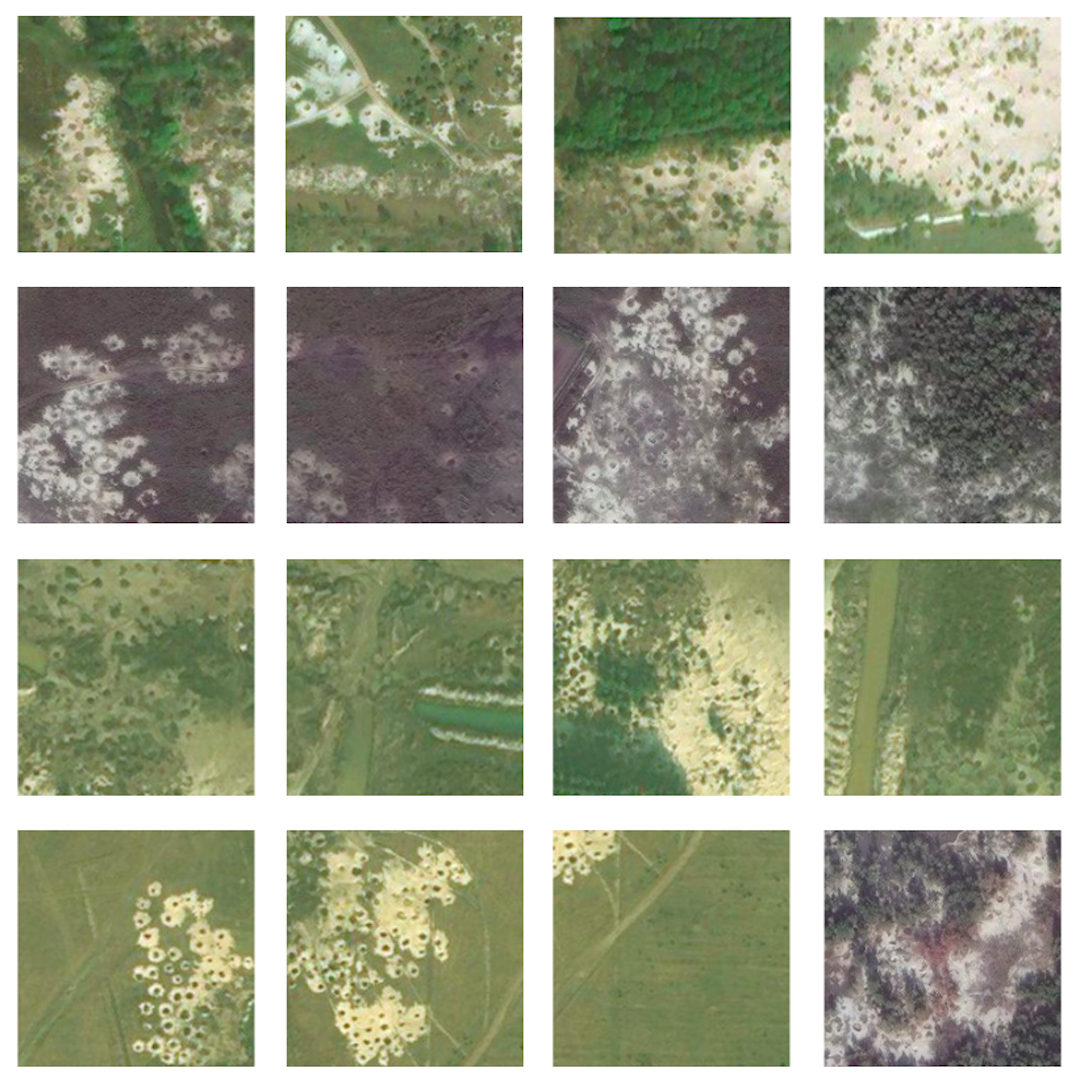
But why was machine learning the right tool to find the information that Texty was looking for?
Classical programming requires you to specify step-by-step instructions for the computer to follow. While this approach works for solving a wide variety of problems, it isn't up to the task of recognising examples of illegal amber mining in a huge amount of satellite images. There are just so many visual elements that the computer would need to consider that it's impossible to come up with a step-by-step set of rules that could teach the software to distinguish between real examples of illegal amber mining and things that might just look similar to it.
Fortunately, machine learning systems are well-positioned to solve this problem.
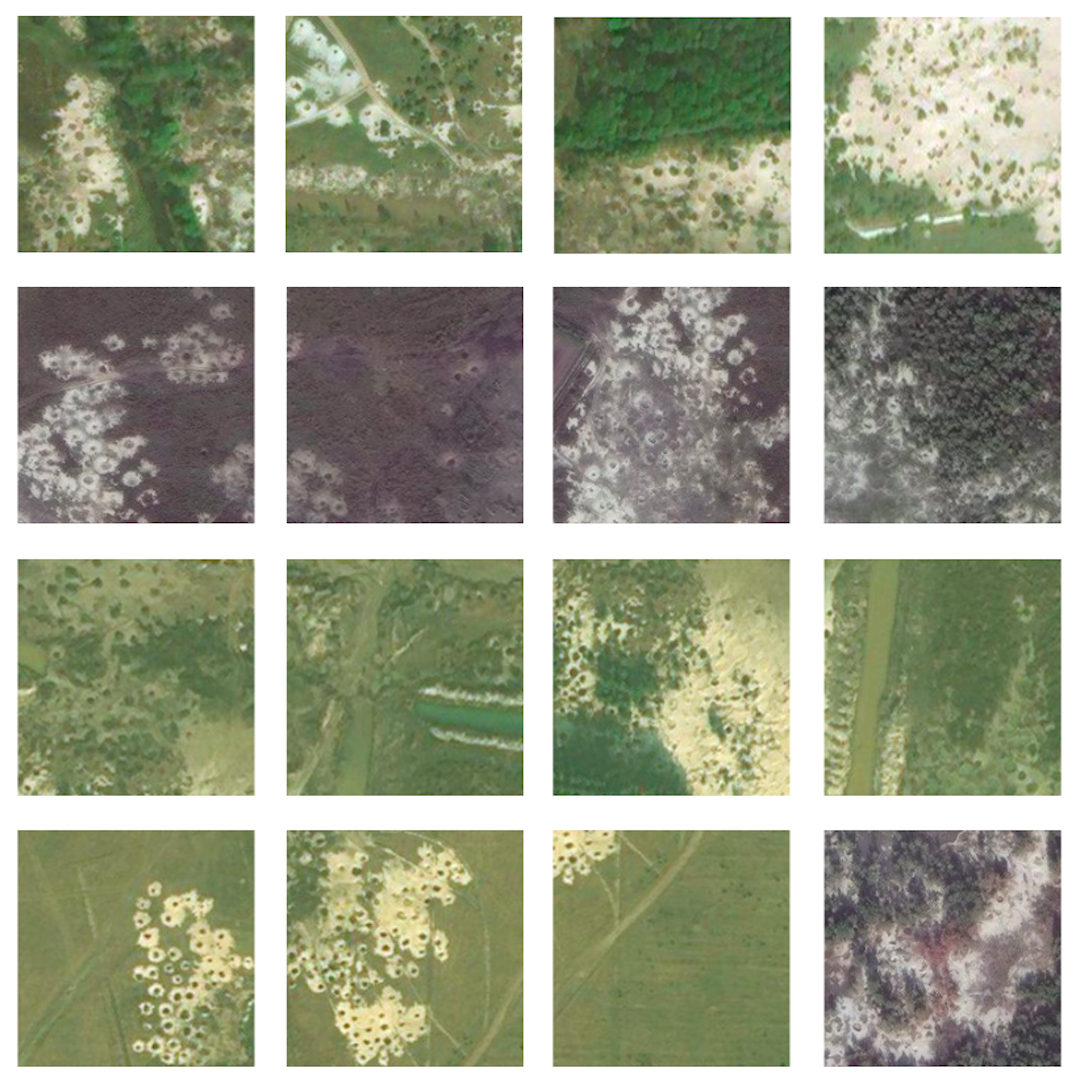
Focus on the process
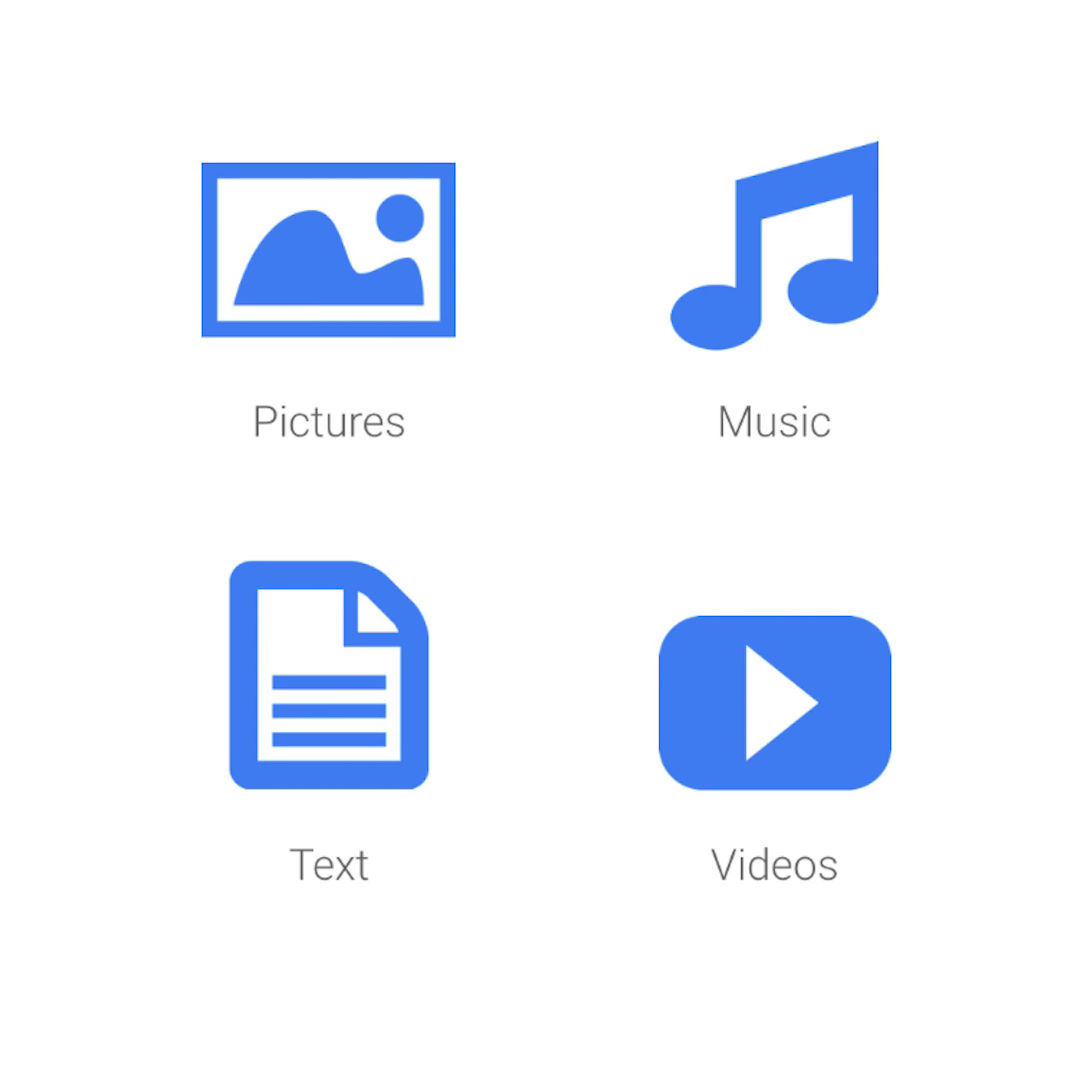
Keep in mind that what you will learn in this course – how to spot illegal amber mining – is only one example. Following the same process, machine learning can be used to perform a number of different journalistic tasks and can even be applied to analyse different types of content, not only images. We will review some other use cases at the end of the course. As we go through the exercise, remember to focus on the process rather than on the specific case study.
Now, before we start the actual exercise, we need to dedicate a few minutes to meeting and setting up the tool we will learn to use in the next lessons: Google Cloud AutoML Vision.
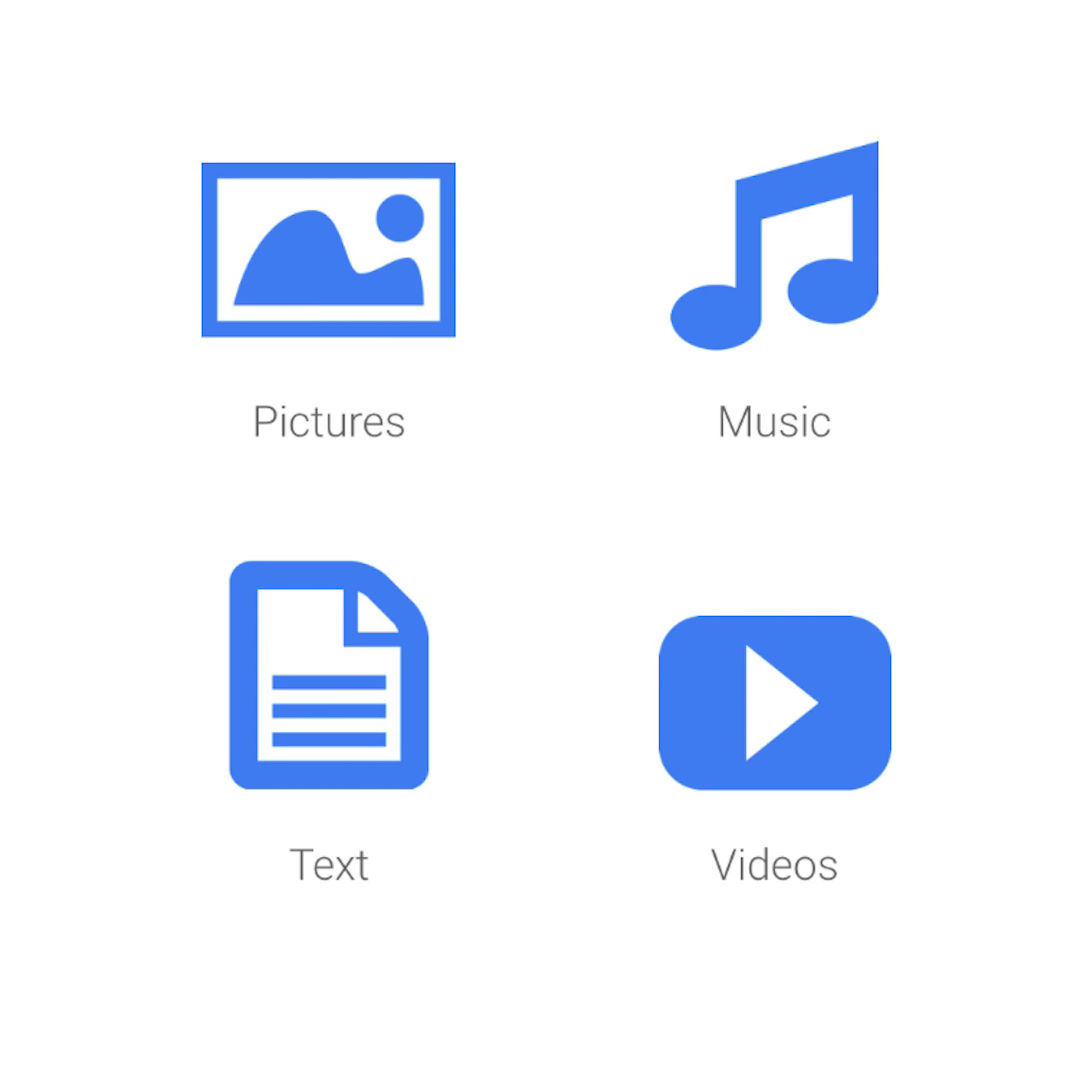
-
-
-
Machine Learning, journalism and you
Bài họcHow machine learning is entering your personal and professional life.