What is Machine Learning
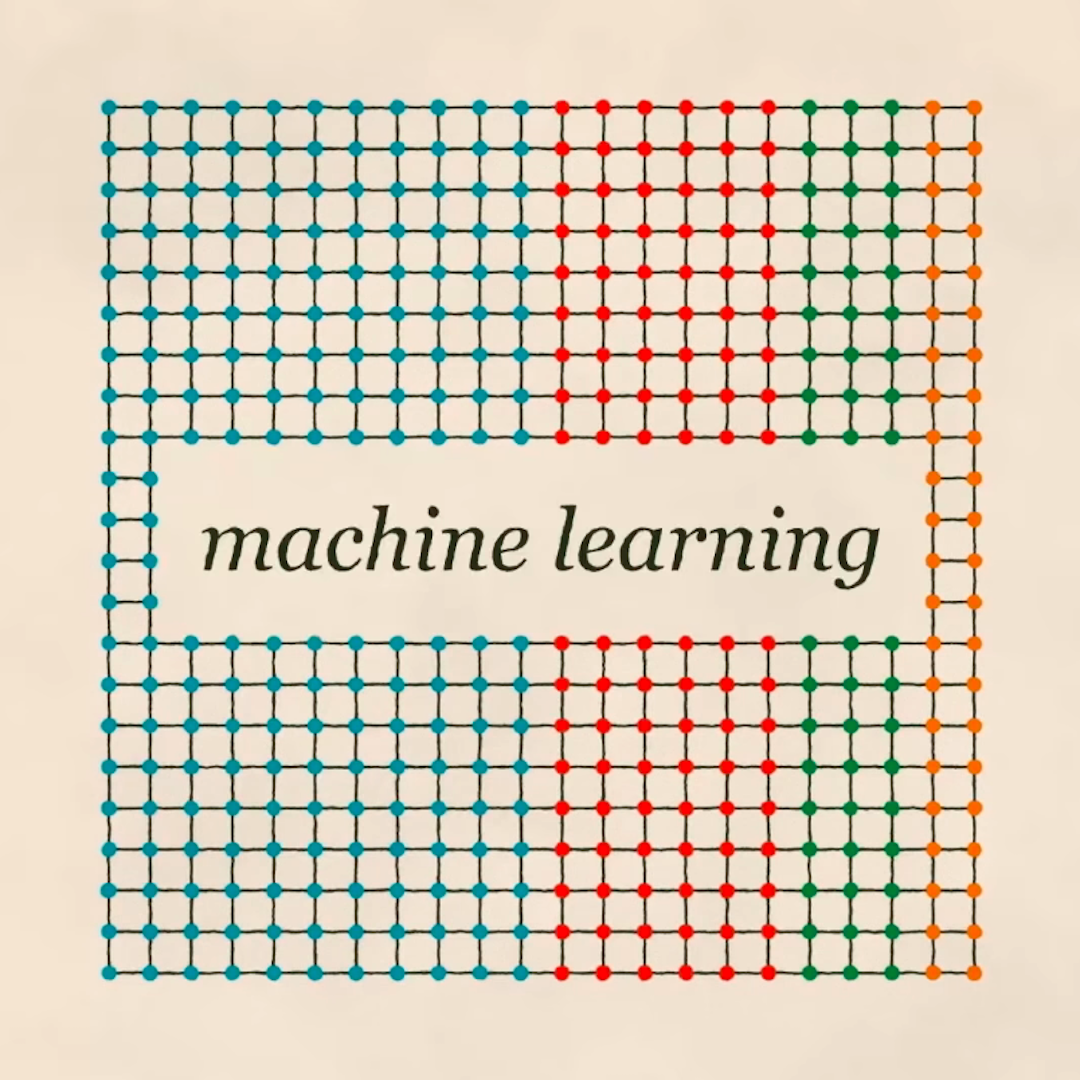
Machine Learning for journalists. What you will learn in this course.
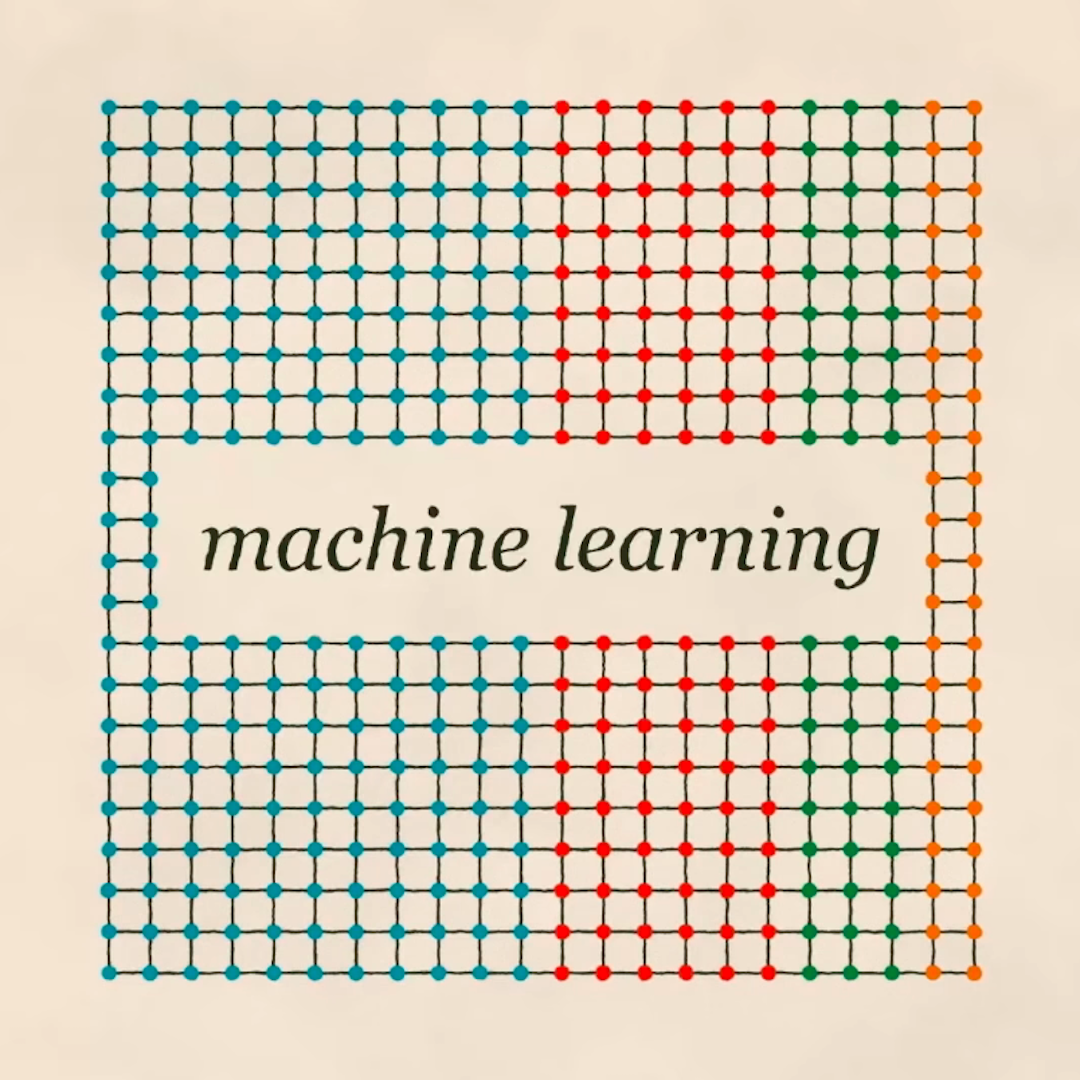
Journalism and Machine Learning
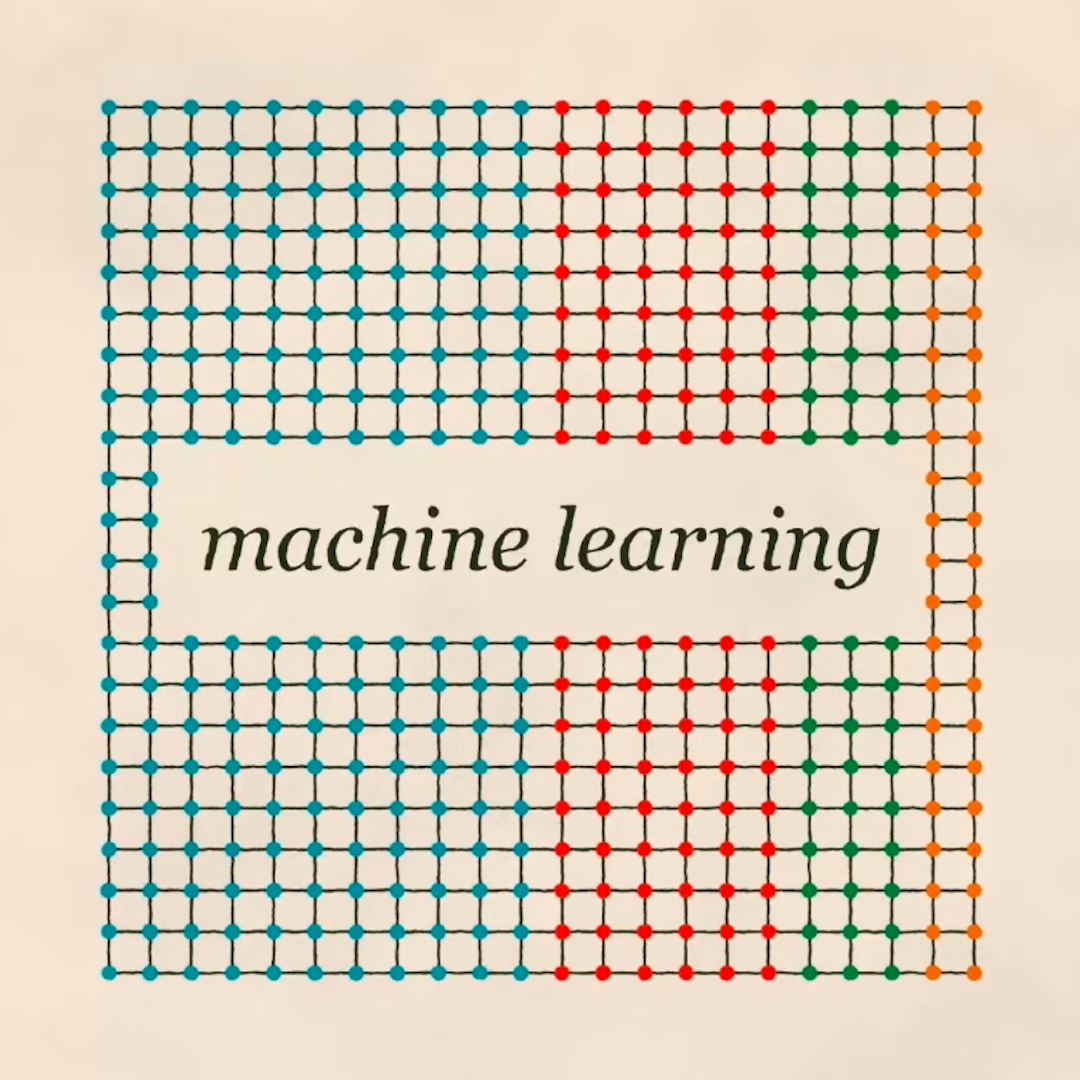
How can journalists use machine learning (ML) to enhance their journalistic work? This is the question that we will explore in this course.
The course will help you understand what are the situations when machine learning is the right tool to support your reporting and will teach you how to train a machine learning model.
This is a follow-up to the course Introduction to Machine Learning. If you haven't taken it yet, we encourage you to do so before proceeding with this course.
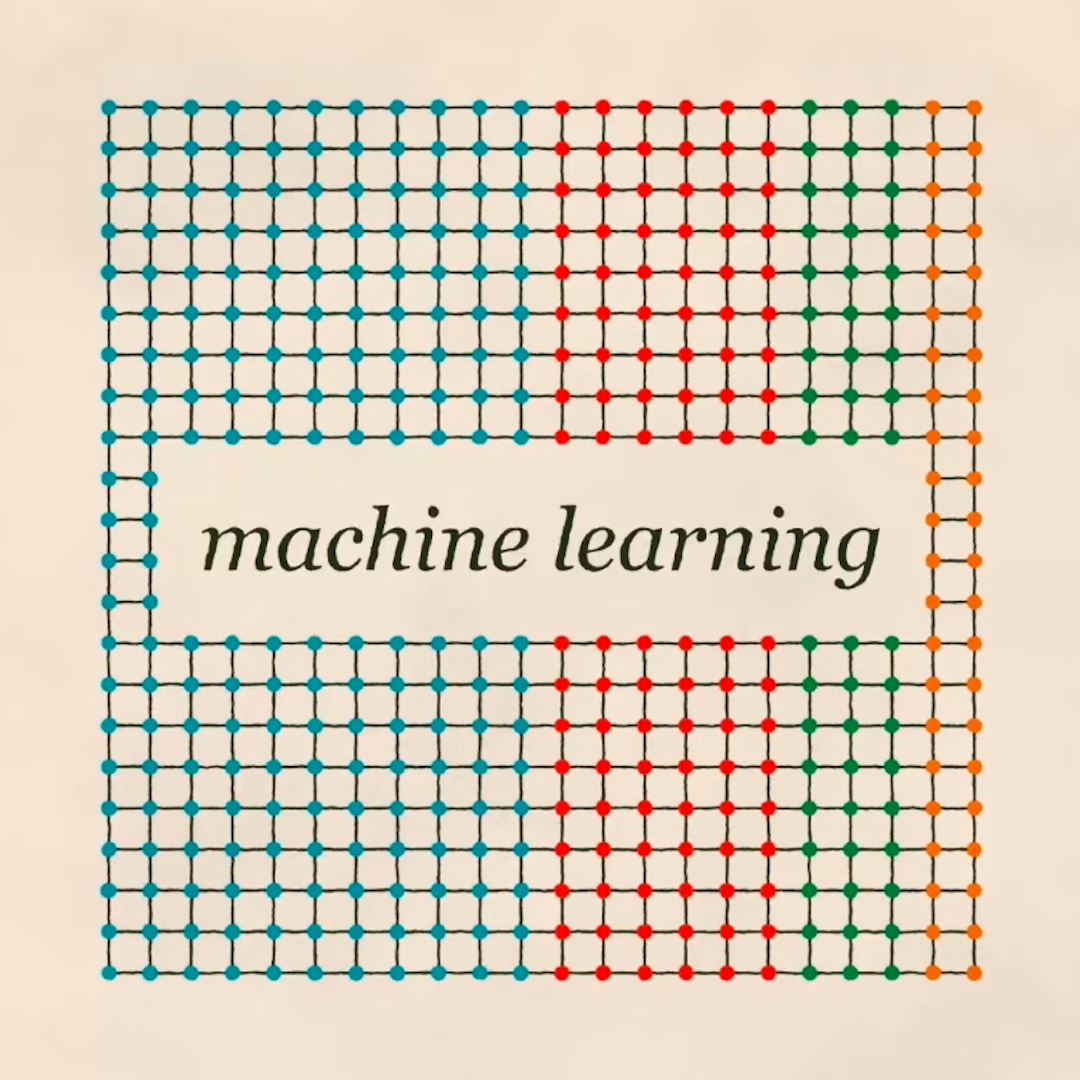
What you can expect from the course
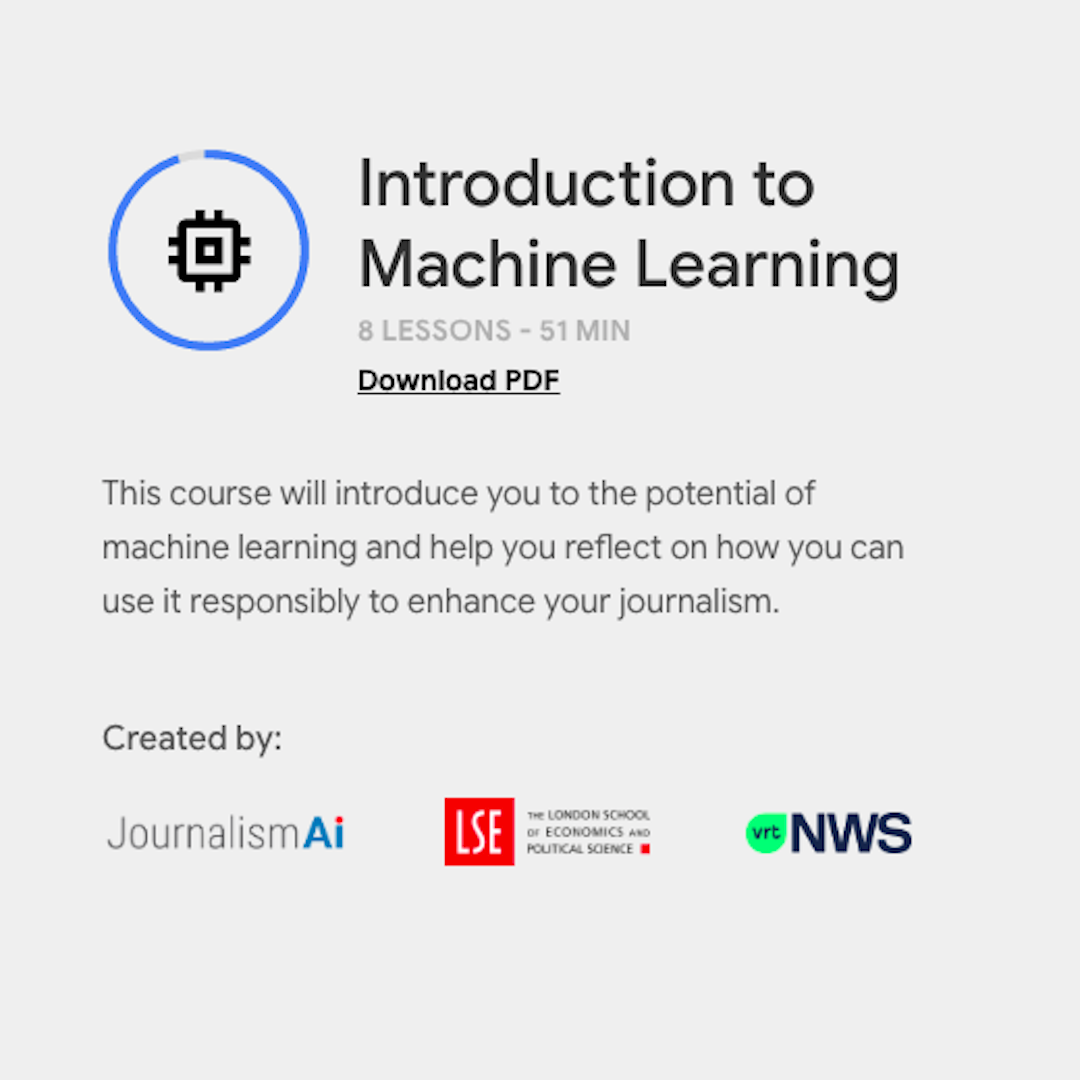
Introduction to Machine Learning explores the potential offered by machine learning to news organisations and explains how journalists can use it responsibly to enhance their reporting.
This course wants to go one step further and show, via a real-life example that we will introduce in the next lesson, what results journalists can achieve by using machine learning. If you want to learn how machine learning works in practice and how you can use it to report your stories, this course is for you.
Will you be an expert machine learning designer and data scientist at the end of this course? No, sorry. But you will learn the steps that underline how most machine learning processes work and you will be able to run experiments on your own.
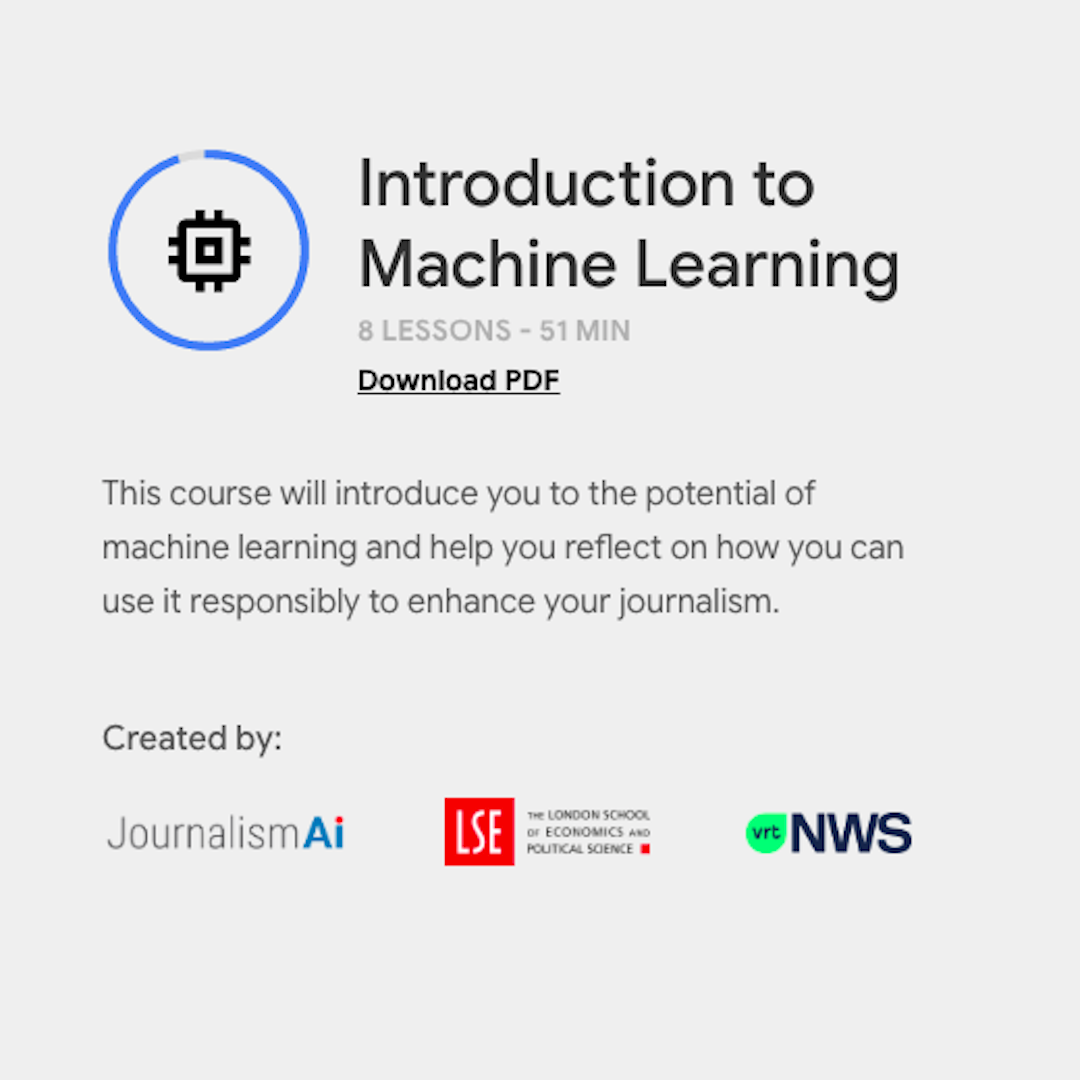
Defining Machine Learning
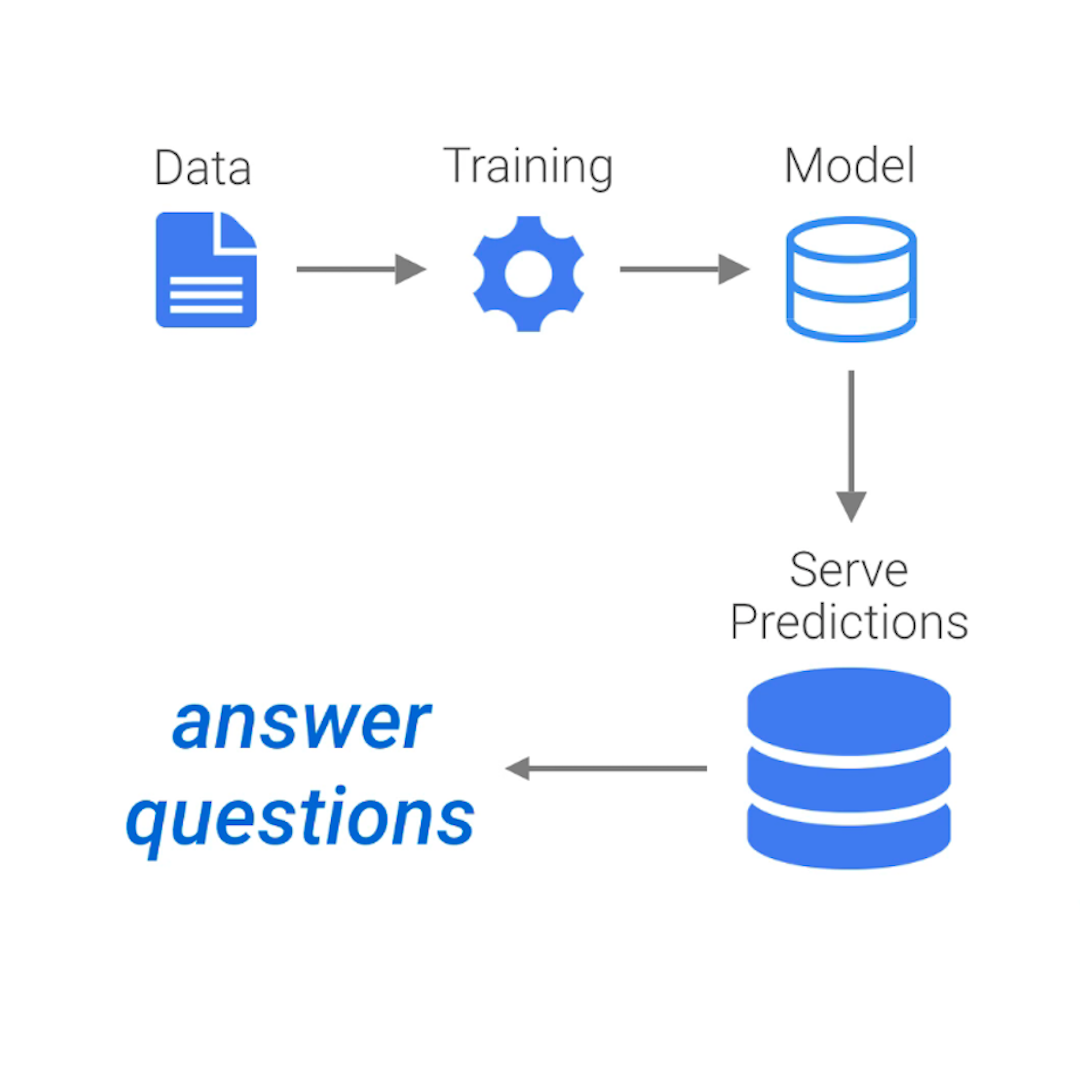
Before we continue, let's make sure we know what we are talking about. What is machine learning?
Machine learning is part of a collection of technologies that are grouped under the umbrella term "artificial intelligence" (AI). As with most of the terminology in the field of artificial intelligence, there is no unique definition of machine learning.
In simple terms, machine learning is a technology that uses data to answer questions. More formally, it refers to the use of algorithms that learn patterns from data and are able to perform tasks without being explicitly programmed to do so.
Moreover, a defining feature of machine learning systems is that they improve their performance with experience and data. In other words: they learn.
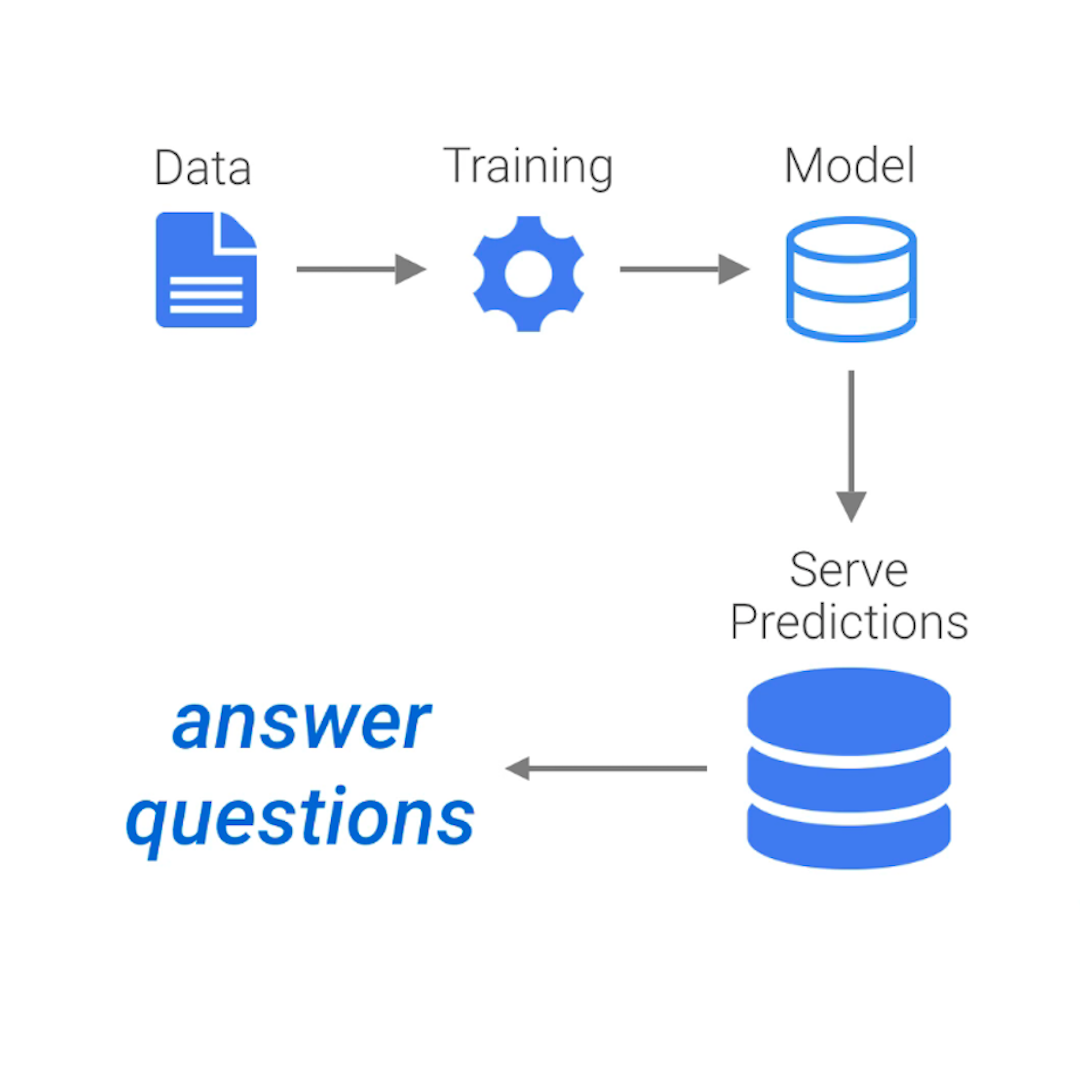
There are various ways to learn
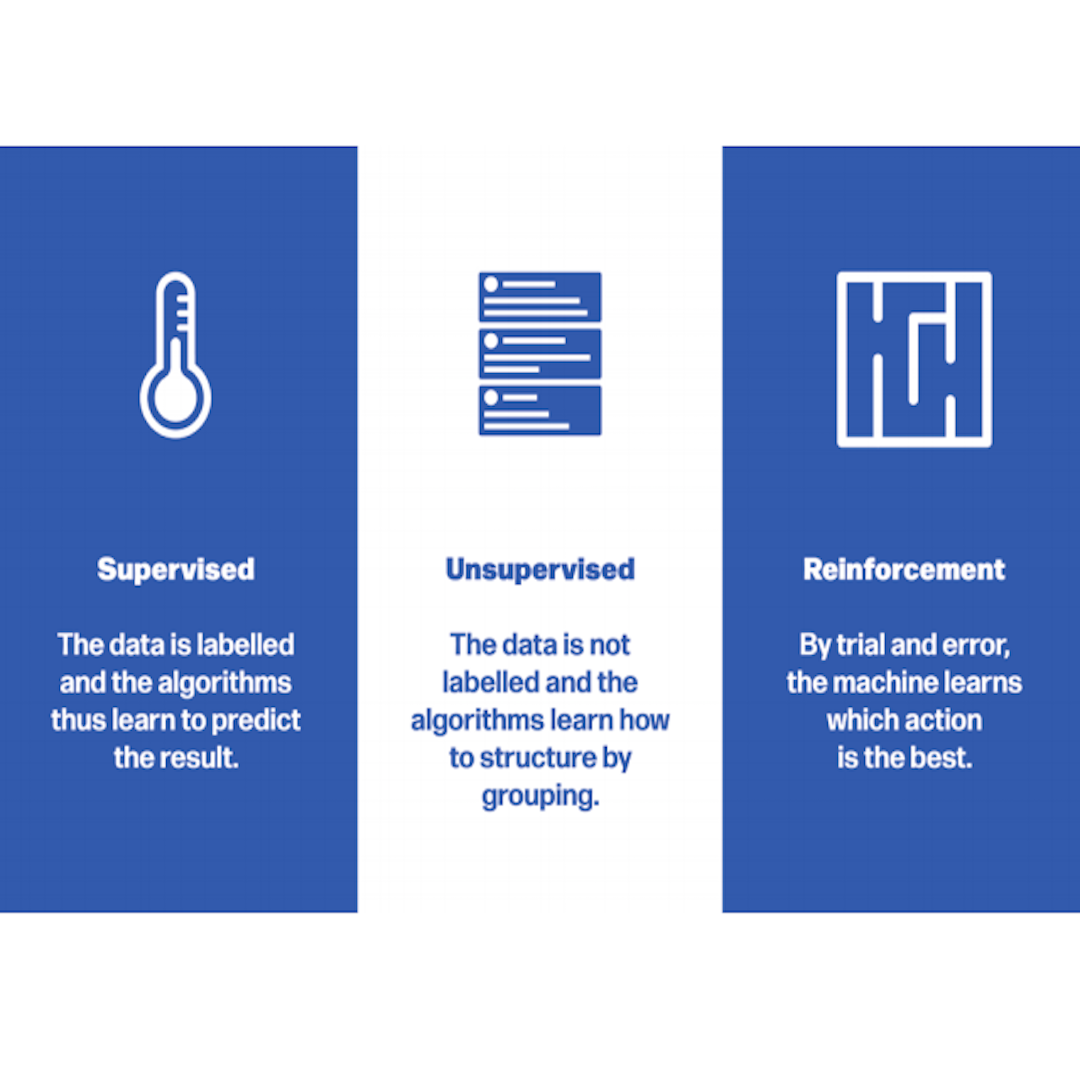
You should keep in mind that there is not one only way for a machine to learn. Different approaches to machine learning are commonly distinguished by the kinds of problems they try to solve, as well as the type and amount of feedback provided.
Broadly, we can divide machine learning into three subareas: 1) Supervised learning; 2) Unsupervised learning; 3) Reinforcement learning. Review the Introduction to Machine Learning to find out more about what differentiates these three categories.
For the purpose of this course, we will focus on supervised learning. This means that we will use labelled examples to train an algorithm to automatically assign the correct label to each new example we will ask it to analyse.
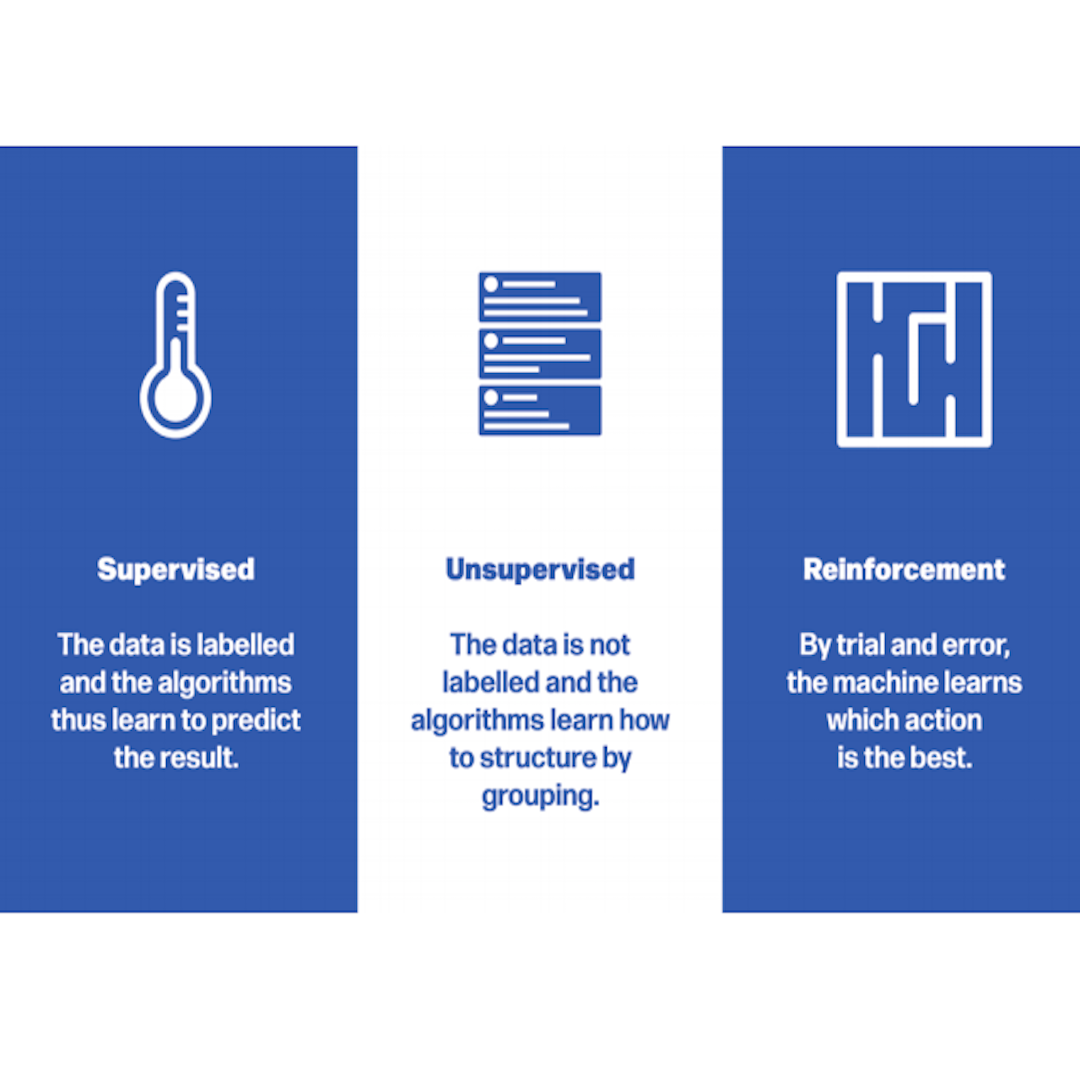
Exploring the potential of Machine Learning
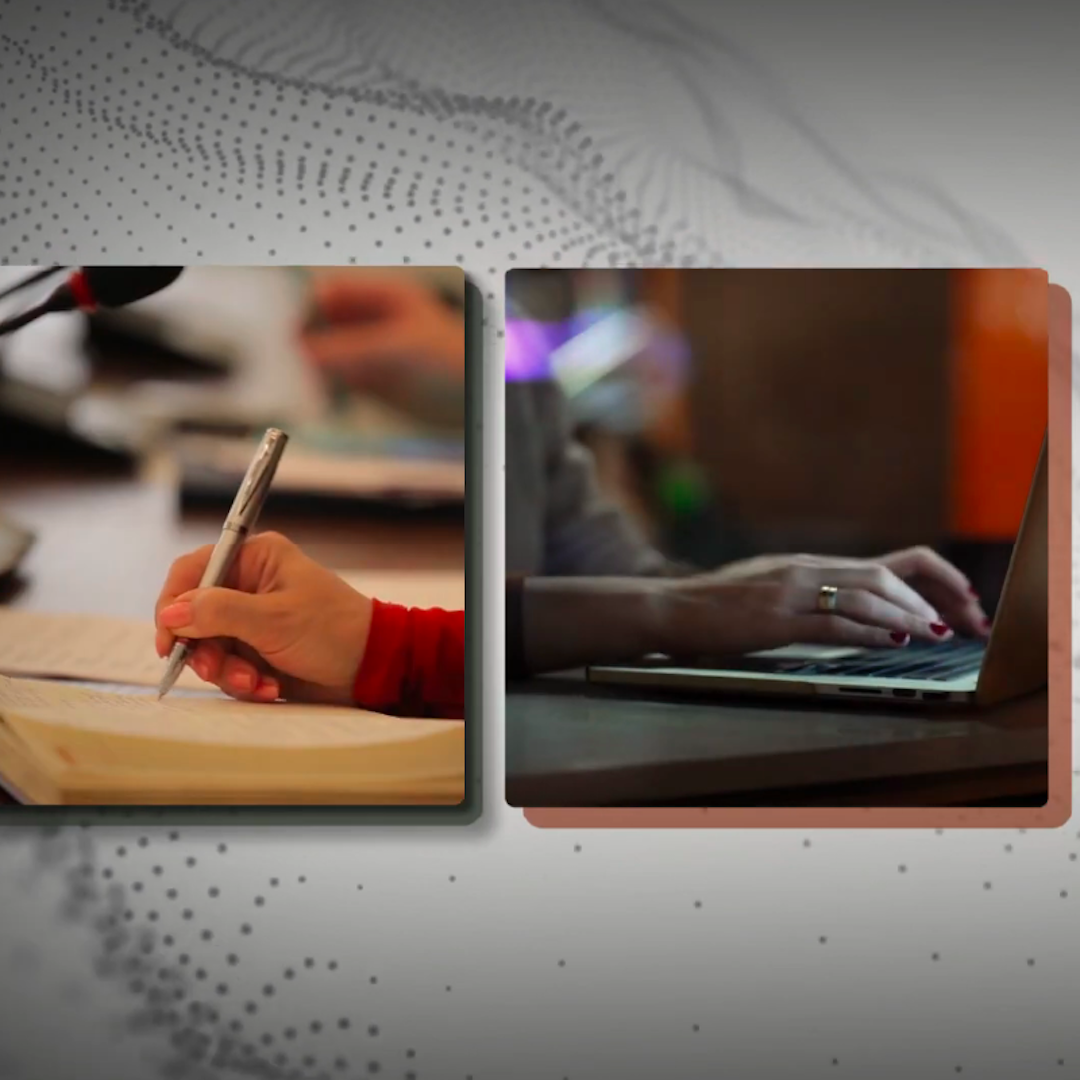
Now that we have reviewed the basics, we are ready to wrap up this introduction and move on.
In the next two lessons, we will introduce a case study that will ground our exercise in a concrete journalistic example and the algorithm that we will use to understand the dynamics behind most machine learning processes.
The following lessons will then focus on the practical step-by-step guide: How to source and prepare the data, how to train your machine learning model and how to test and evaluate its performance.
The last lesson will summarise the key learnings, help you understand how to apply them in your day-to-day reporting and recommend other resources you can use to dive even deeper into the world of machine learning.
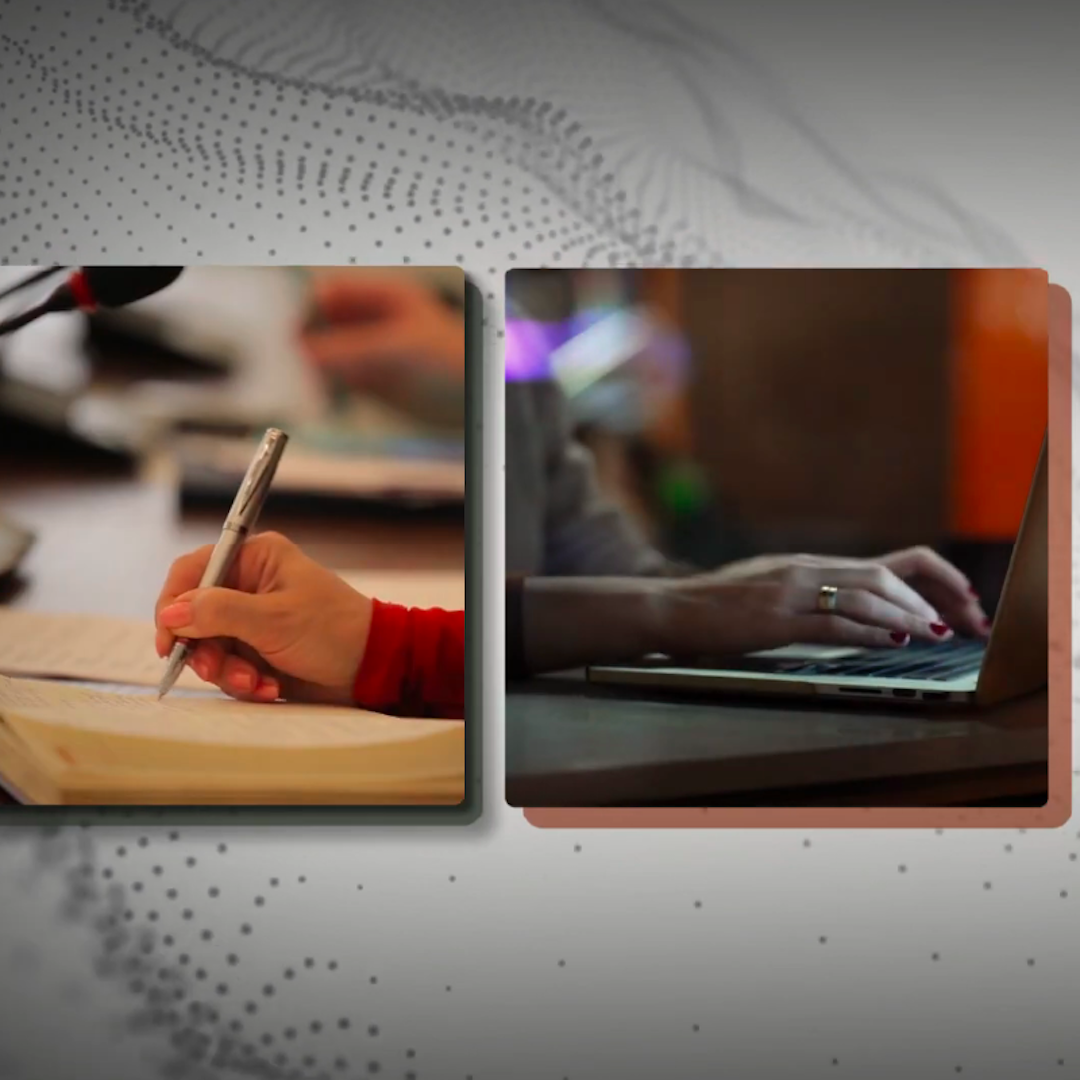