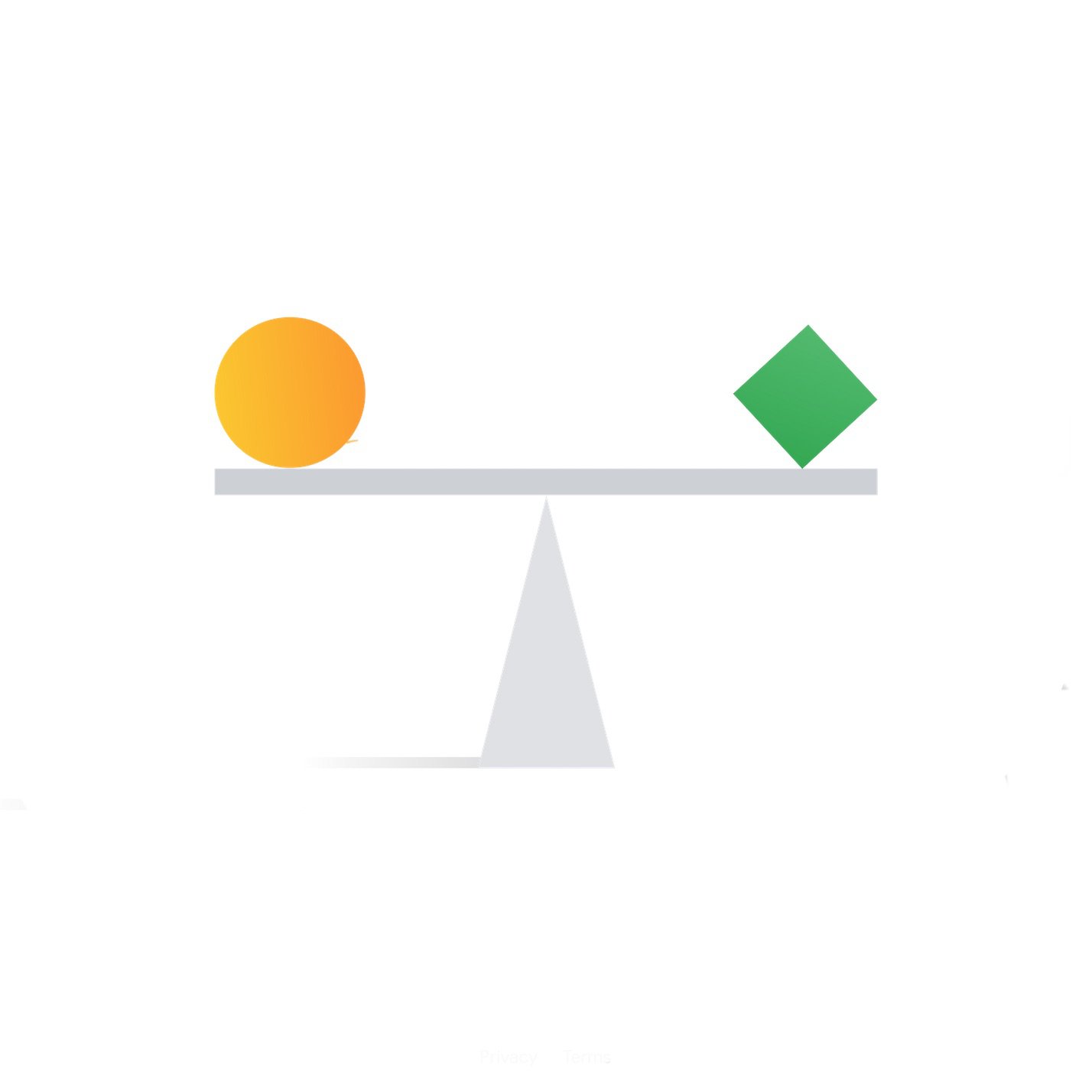
Fairness in Machine Learning
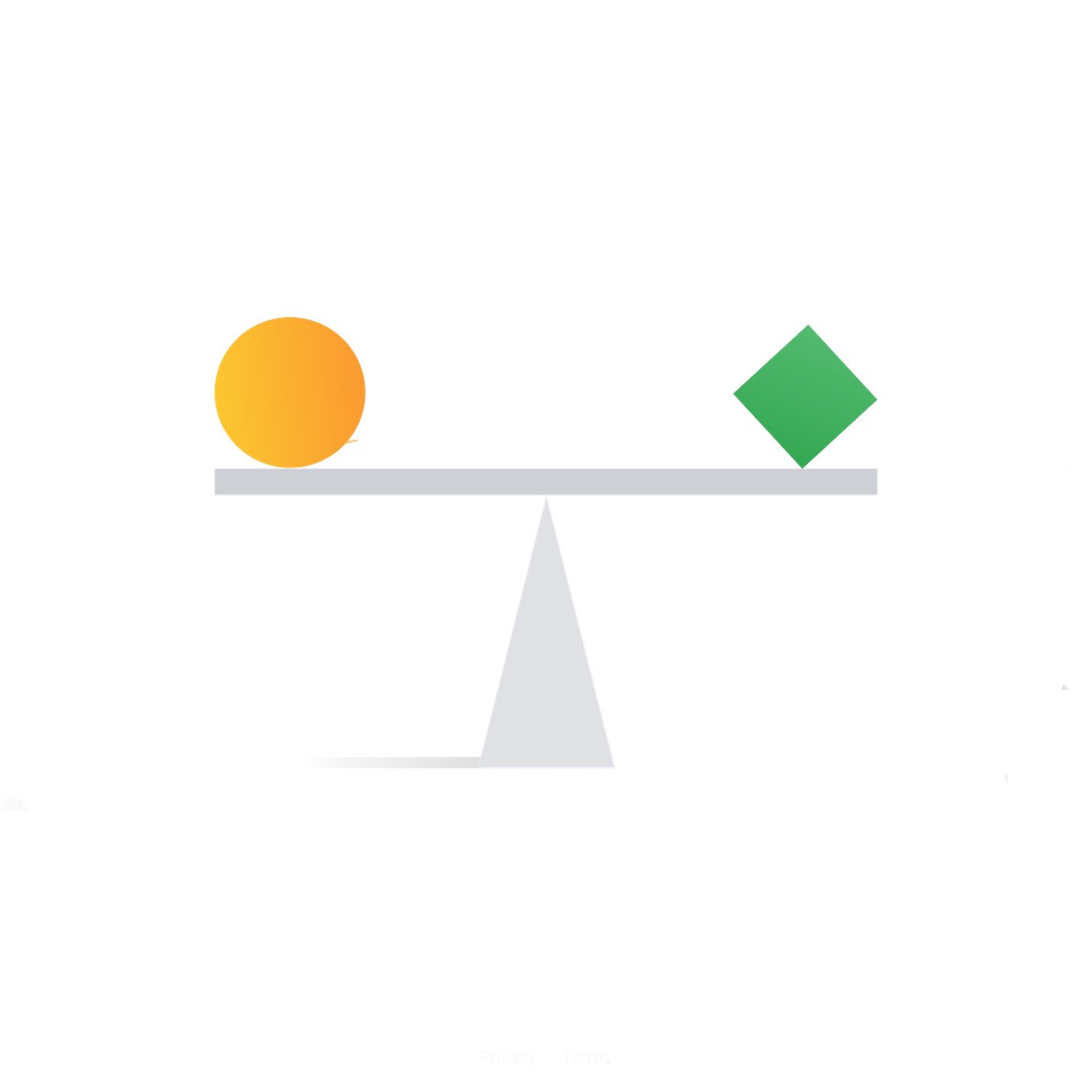
So far, this course showed how machine learning can enhance your work, from saving precious time on existing tasks to opening up new opportunities. ML can do a lot for you, but it comes with challenges you shouldn't overlook.
To address those challenges, a growing number of researchers and practitioners focus on the topic of "fairness" in machine learning. Its guiding principle is that ML should equally benefit everyone, regardless of the societal categories that structure and impact our lives.
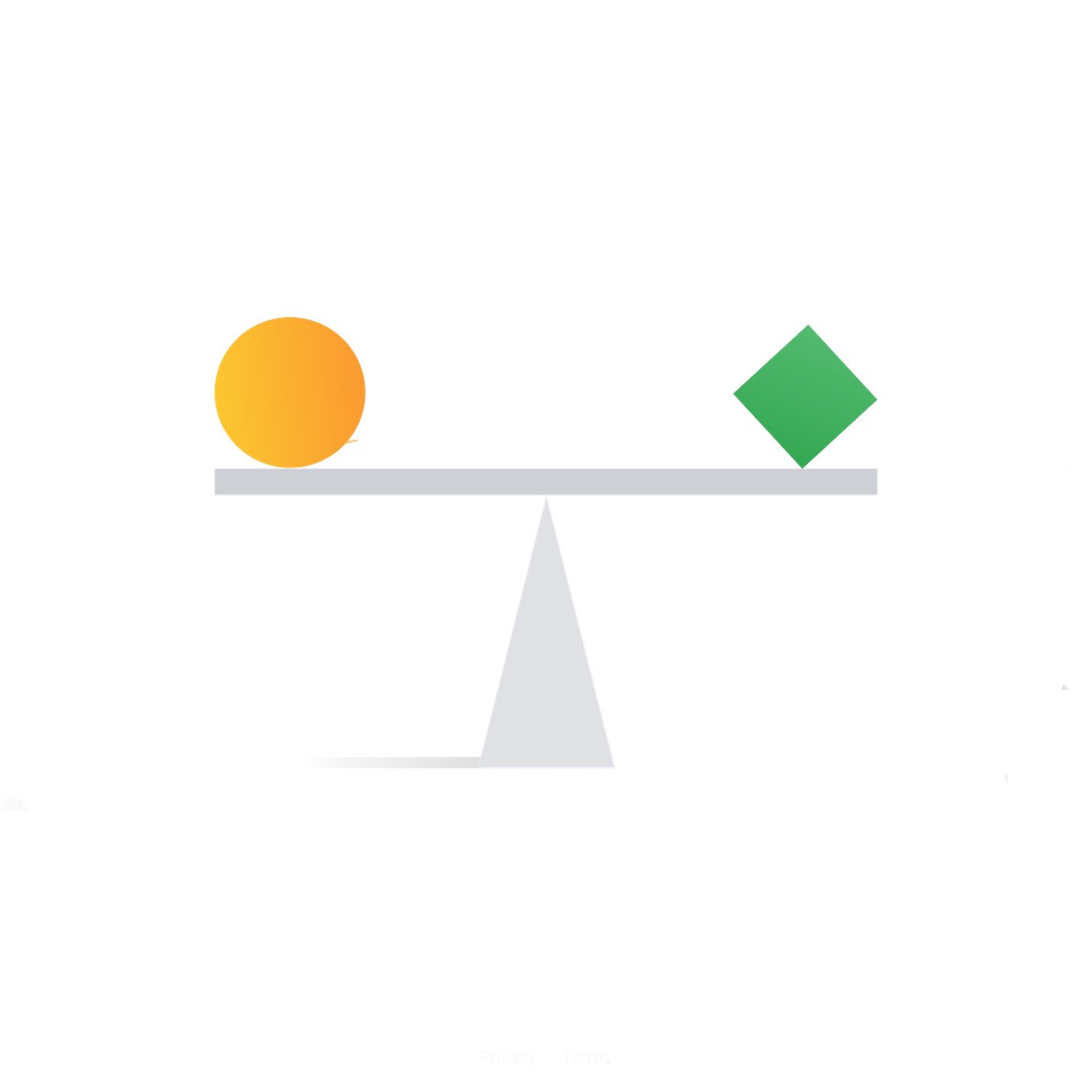
What is bias?
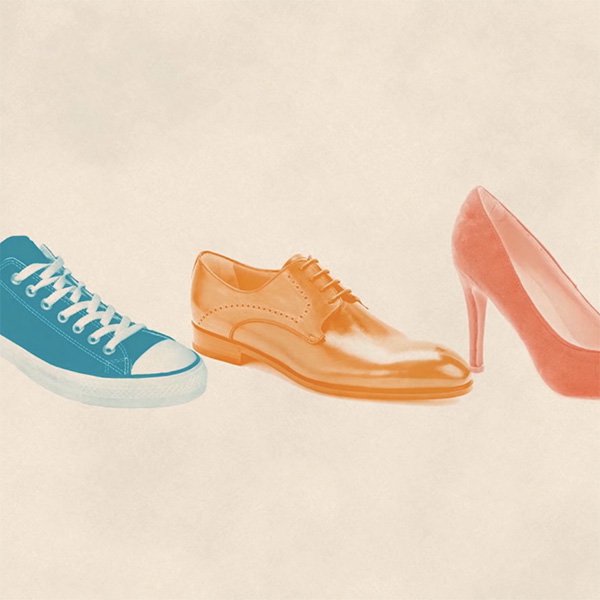
What are the negative consequences that might derive from the use of machine learning? The short answer is: Bias.
As humans, we all have our biases. They are tools our brain uses to deal with the information that is thrown at it every day.
Take this example: close your eyes and picture a shoe. Most likely you pictured a sneaker. Maybe a leather men's shoe. It's less likely that you thought of a high-heeled women's shoe. We may not even know why but each of us is biased toward one shoe over the others.
Now imagine that you want to teach a computer to recognise a shoe. You may end up exposing it to your own bias. That's how bias happens in machine learning. Even with good intentions, it's impossible to separate ourselves from our own biases.
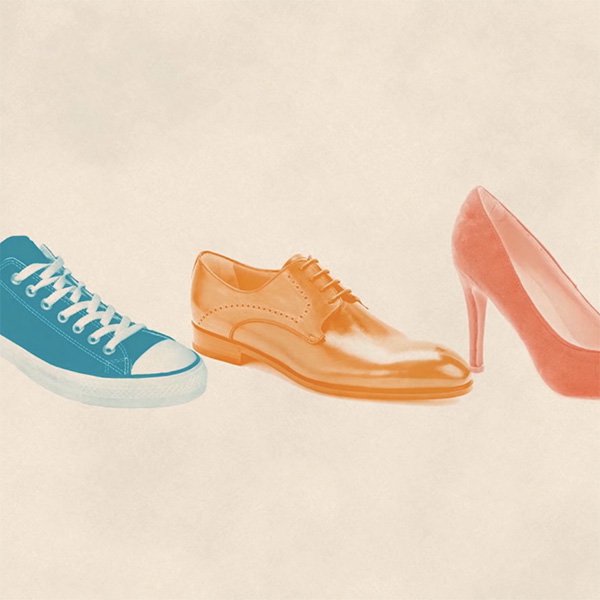
Three types of bias
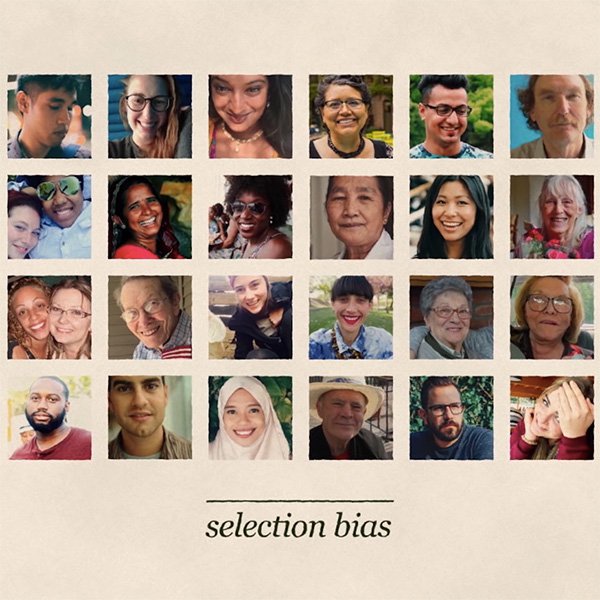
There are different ways in which our own biases risk to become part of the technology we create:
Interaction bias
Take the example before: if we train a model to recognise shoes with a dataset that includes mostly pictures of sneakers, the system won't learn to recognise high heels as shoes.
Latent bias
If you train a ML system on what a scientist looks like using pictures of famous scientists from the past, your algorithm will probably learn to associate scientists with men only.
Selection bias
Say you're training a model to recognise faces. If the data you use to train it over-represents one population, it will operate better for them at the expense of others, with potentially racist consequences.
So what can we do to avoid these biases?
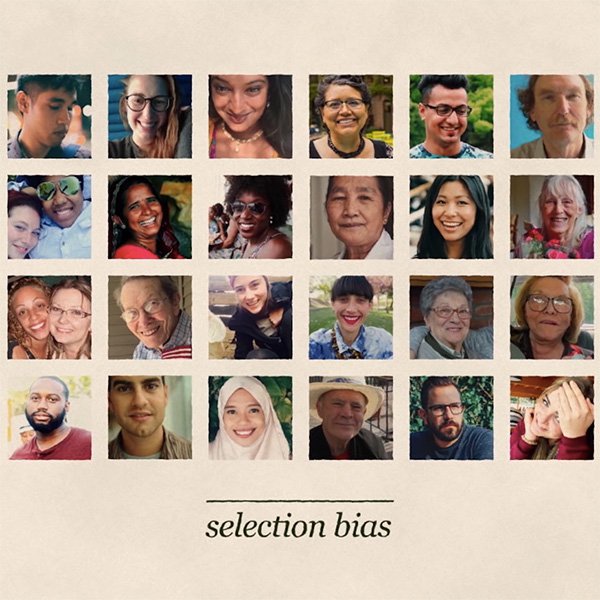
Asking the right questions to avoid bias
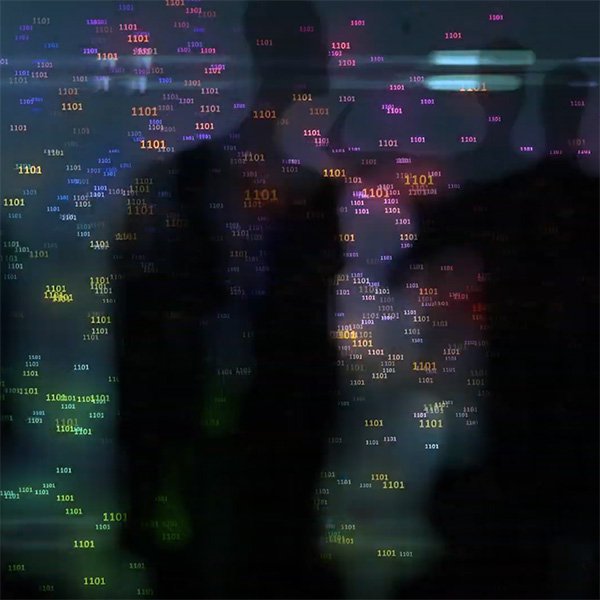
As a journalist, a first line of defence against bias is firmly within your reach: the same values and ethical principles you apply every day in your profession should extend to assessing the fairness of any new technology that is added to your toolbox. Machine learning is no exception.
Furthermore, in all cases you should start by considering whether the consequences might negatively impact individuals’ economic or other important life opportunities. This is critical especially if the data you use includes sensible personal information.
Often, the unfair impact isn't immediately obvious, but requires asking nuanced social, political and ethical questions about how your machine learning system might allow bias to creep in.
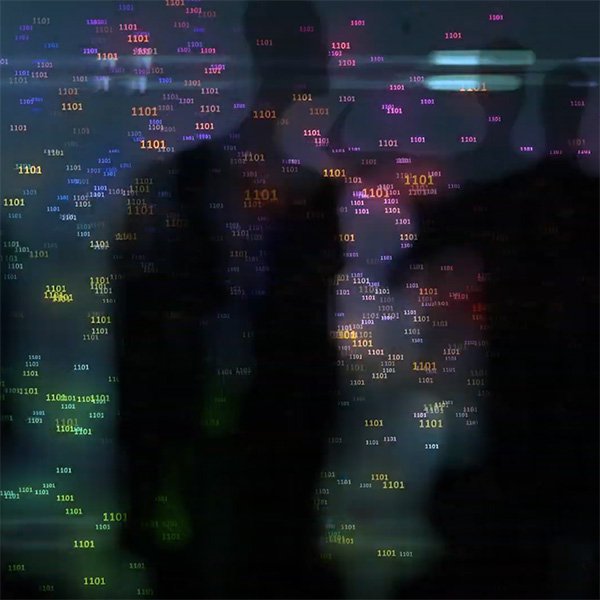
Considering the main sources of bias
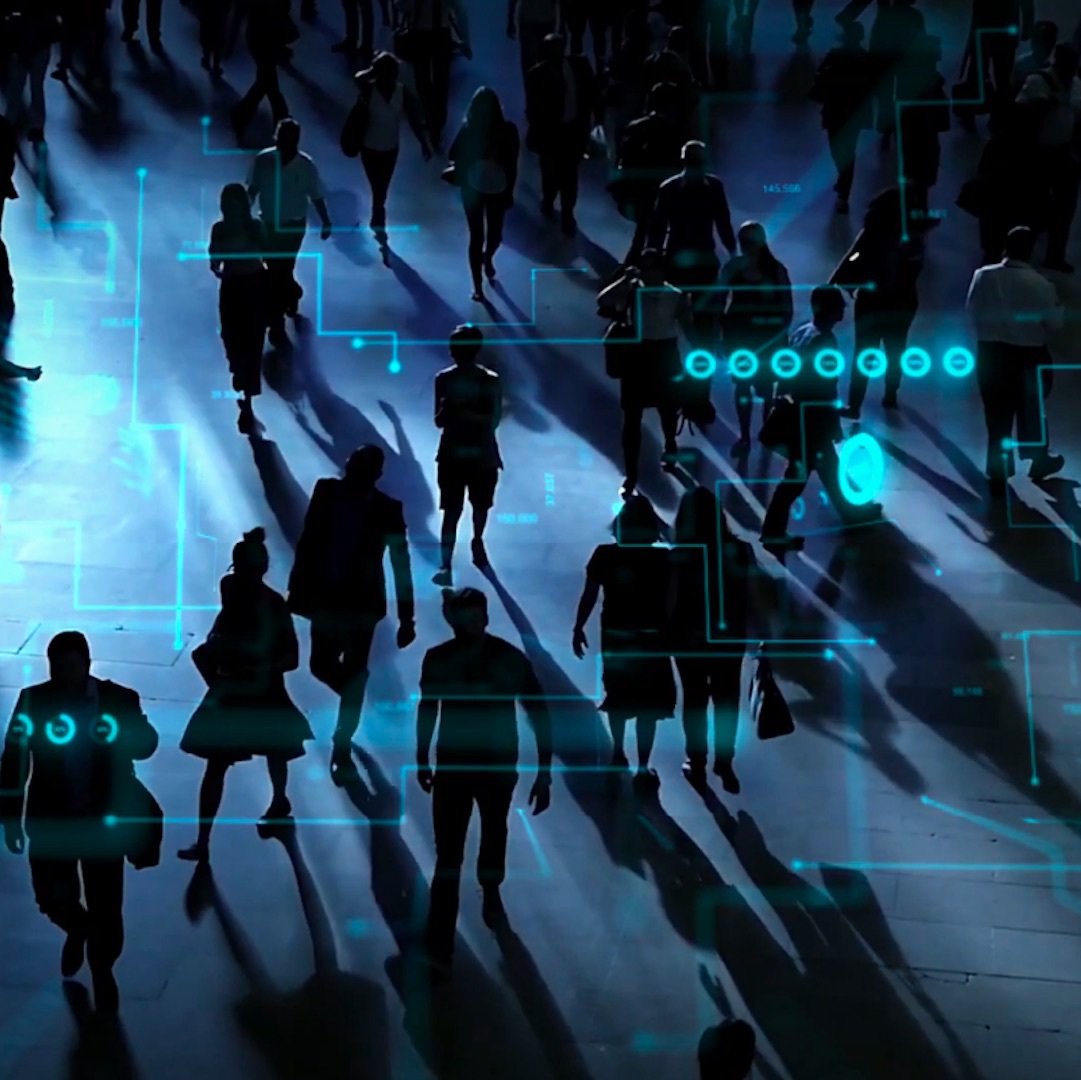
While no training data will ever be perfectly ‘unbiased’, you can greatly improve your chances of building a fair model if you carefully consider potential sources of bias in your data, and take steps to address them.
The most common reason for bias creeping in is when your training data isn't truly representative of the population that your model is making predictions on. You must make sure to have enough data for each relevant group.
A different kind of bias manifests itself when some groups are represented less positively than others in the training data. You should consider reviewing your data before using it to train a model, in order to verify whether it carries any prejudices that might be learned and reproduced by the algorithm.
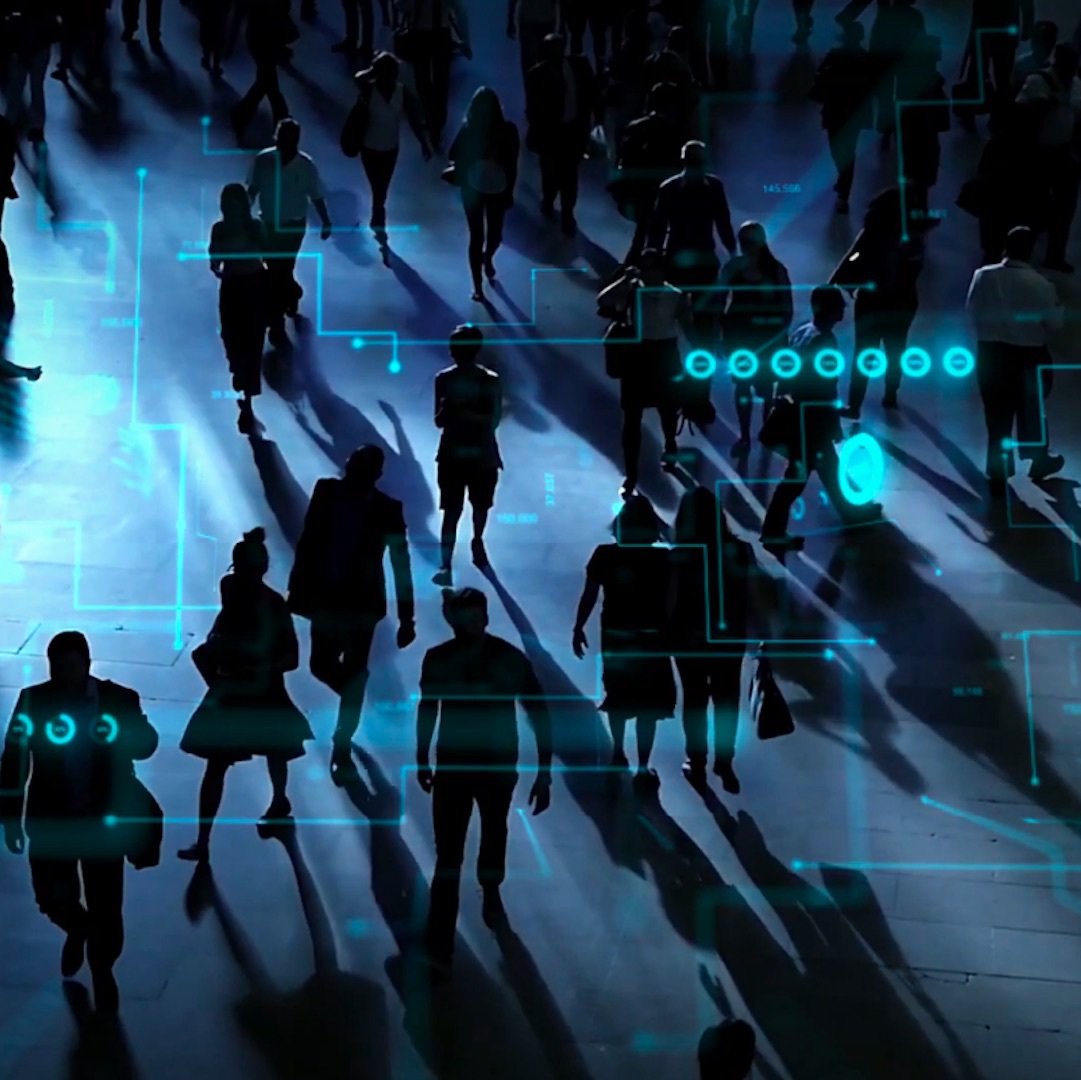
Preventing bias: it starts with awareness
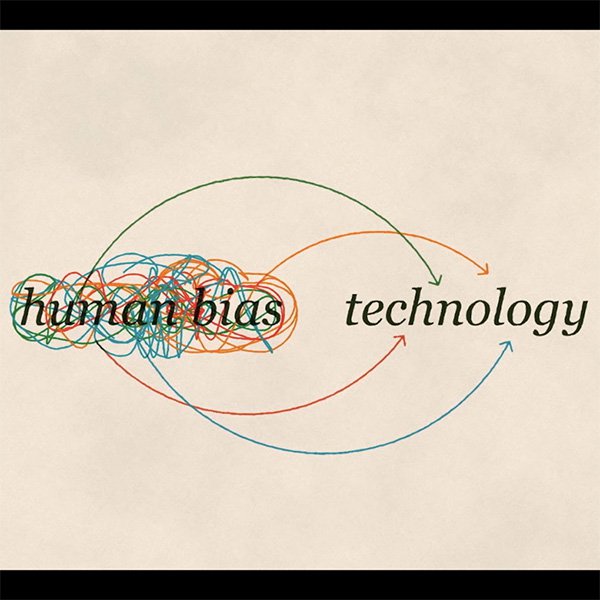
Bias can emerge in many ways: from training datasets, because of decisions made during the development of a machine learning system, and through complex feedback loops that arise when a ML system is deployed in the real world.
Some concrete questions you might want to ask in order to recognize potential bias include:
- For what purpose was the data collected?
- How was the data collected?
- What is the goal of using this set of data and this particular algorithm?
- How was the source of data assessed?
- How was the process of data analysis defined before the analysis itself?
Bias is a complex issue and there is no silver bullet. The solution starts with awareness and with all of us being mindful of the risks and taking the right steps to minimise them.
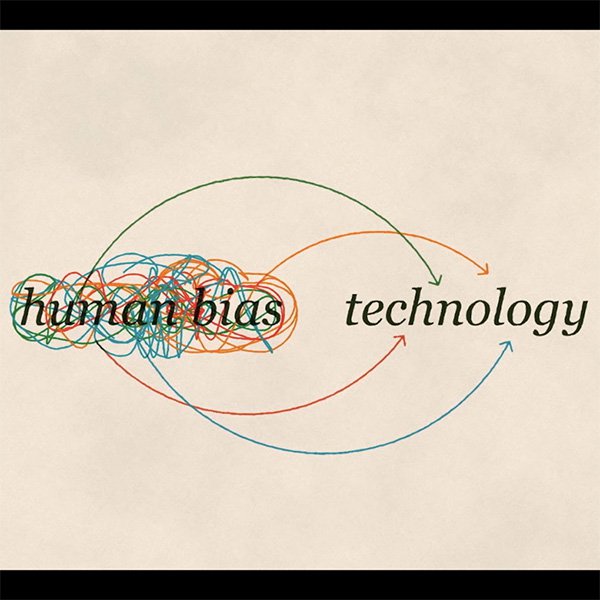
-
Google Translate: Translations on-the-go.
LessonSpeak the language just about anywhere in the world. -
-
Verification: Google Search
LessonMaster advanced search shortcuts to filter results by date, relevance and language.